Towards MR contrast independent synthetic CT generation.
PO-1698
Abstract
Towards MR contrast independent synthetic CT generation.
Authors: Attila Simko1, Mikael Bylund2, Gustav Jönsson1, Tommy Löfstedt3, Anders Garpebring1, Tufve Nyholm1, Joakim Jonsson1
1Umeå University, Department of Radiation Sciences, Umeå, Sweden; 2Umeå University, Departmen of Radiation Sciences, Umeå, Sweden; 3Umeå University, Department of Computing Science, Umeå, Sweden
Show Affiliations
Hide Affiliations
Purpose or Objective
The use of synthetic CT (sCT) would reduce the costs and scan time while improving the accuracy of organ delineations in the radiotherapy workflow. The performance of deep learning (DL) solutions for sCT generation is steadily increasing, however most proposed methods were trained and validated on private datasets from a single scanner with the same acquisition settings for each patient. Such solutions might not perform equally well on other datasets, limiting their general usability and therefore value.
Material and Methods
The generalization is best improved through a more varied dataset, however this might be impossible to achieve due to the increased price, complexity or other limitations. As an alternative, we propose to incorporate a DL model trained on a wide range of MR contrast as a pre-processing step by training a model for sCT generation using artificial PD, T1 and T2 maps. Using a dataset of only T2w MR images, the robustness of this approach is compared to a model that was trained using the MR images directly.
The difference between the two approaches is shown on the low resolution figure below.
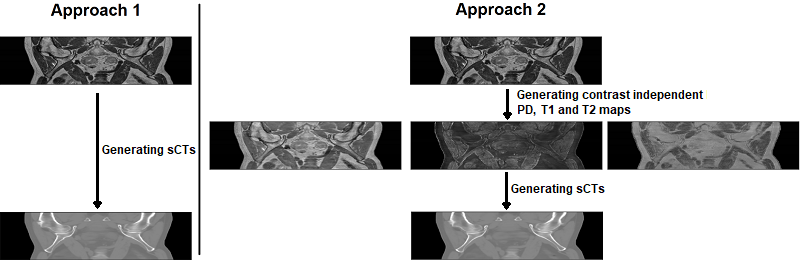
Results
On T2w images acquired using the same settings as for the training dataset, both models perform similarly well. While the baseline model performs best on T2w images, our proposed robust method shows no significant change in performance between T1w and T2w contrasts, evaluated on images from three different scanners. Evaluating on five different contrast and a wide range of synthetic contrast, the robustness of our proposed method is further underlined. As a more functional evaluation method, we show that our proposed robust method also performs well with regards to radiological depth calculations.
Conclusion
We have propagated the robustness of a machine learning solution, by using it as a pre-processing step when training a model for sCT generation. The results show that imbalanced datasets can benefit from synthetic data augmentation, without sacrificing performance on real data. Our code and trained models are also made publicly available.