Iterative reconstruction of MVCT with deep neural networks
PO-1687
Abstract
Iterative reconstruction of MVCT with deep neural networks
Authors: Sho Ozaki1, Shizuo Kaji2, Kanabu Nawa3, Toshikazu Imae3, Keiichi Nakagawa3
1Hirosaki University, Graduate School of Science and Technology, Hirosaki, Japan; 2Institute of Mathematics for Industry, Kyushu University, Division for Intelligent Societal Implementation of Mathmatical Computation, Fukuoka, Japan; 3University of Tokyo Hospital, Department of Radiology, Tokyo, Japan
Show Affiliations
Hide Affiliations
Purpose or Objective
Deep learning is widely used for image quality enhancement in medical imaging. However, deep learning-based methods usually need a large amount of training data,
and collecting such big data is often expensive for the medical staff. Deep image prior (DIP) gives one of the solutions; it can improve image quality without training data. By extending the DIP, we propose a novel method of CT reconstruction with deep neural networks. We apply our methods to MVCT reconstruction, which is used in helical tomotherapy.
Material and Methods
Iterative reconstruction (IR) solves the inverse problem by minimizing a loss function. Often regularization terms such as the total variation (TV) are introduced to incorporate prior on the images. Instead of optimizing the voxel values directly, the DIP can optimize the parameters of a convolutional neural network (CNN) whose output is the voxel values. This indirect optimization can be thought of as an application of DIP for CT reconstruction, where the output image and the observed projection are taken in a consistency loss function. Our primary contribution is an optimization methodology for the DIP reconstruction where we start with large weights of low-resolution layer terms in the loss function, and then progressively increase the weights of high-resolution layer terms with an increasing iteration, as visualized in Figure 1. Our method stabilizes the optimization of model parameters, and improves high-resolution image quality.
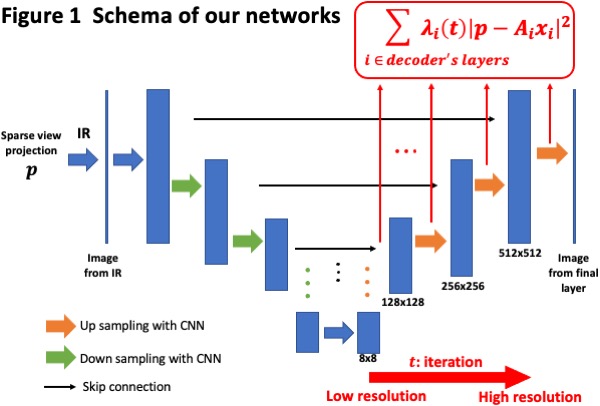
Results
We demonstrate the effectiveness of our method with MVCT reconstruction. We reconstruct full scan MVCT which is clinically used in tomotherapy, and spare view (1/10-view projection) MVCT with 1) IR, 2) IR+TV, 3) DIP reconstruction, and 4) the proposed method. We evaluate image quality by using SSIM and PSNR with a full scan MVCT image as the ground truth. The results of reconstructed images are shown in Figure 2 with the values of the metrics. The proposed method achieved the best results among 1)~4), while the IR+TV and the DIP are compatible with each other with respect to SSIM and PSNR. Although the IR+TV reduces the artifacts and tends to produce flat regions, the boundaries of tiny structures in the image are blurred. In contrast, our method captures tiny structures of the full scan MVCT image with reducing the artifacts and noises.
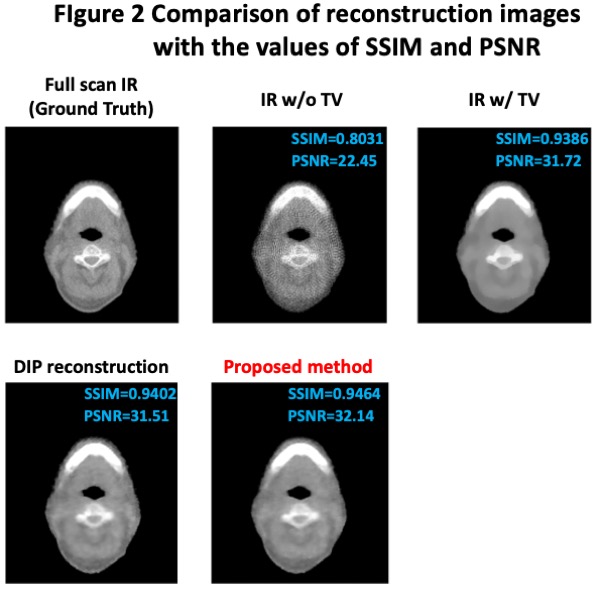
Conclusion
The proposed method improves the image quality of sparse view MVCT images without a large amount of training data. The performance of our method exceeds those of the traditional data-free methods such as the IR+TV and the DIP. This method can be potentially applied to clinical usages such as in dose reduction of MVCT, 4D MVCT, and a fast MVCT sinogram acquisition. Furthermore, our method can be applied to not only MVCT but also CBCT and other CT modalities.