Generation of synthetic radiation pneumonitis images using deep learning
Zhixiang Wang,
The Netherlands
PO-2203
Abstract
Generation of synthetic radiation pneumonitis images using deep learning
Authors: Zhixiang Wang1, Junzhuo Liu2, Ye Zhang2, Zhen Zhang3, Andre Dekker4, Alberto Traverso4
1Maastricht University Medical Centre+, Department of Radiation Oncology (Maastro), GROW School for Oncology, Maastricht, The Netherlands; 2Chongqing University of Posts and Telecommunications, Key Laboratory of Data Engineering and Visual Computing, Chongqing, China; 3 Maastricht University Medical Centre+, Maastro, Maastricht, The Netherlands; 4Maastricht University Medical Centre+, Department of Radiation Oncology (Maastro), Maastricht, The Netherlands
Show Affiliations
Hide Affiliations
Purpose or Objective
Radiation pneumonitis (RP) is a common side effect of thoracic radiation treatment and relevant for patients’ quality of life receiving RT. Researchers are investigating DL methods for RP prediction in planning RT scans. However, the massive data requirements of deep learning have long been a limitation in the field, especially for RP prediction, where it is often difficult (and sometimes impossible) to obtain enough training data to build a robust RP detection algorithm. Until now, there is no study that can generate RP synthetic images to overcome the deficiencies of insufficient data and data imbalance. We proposed a mask self-attention mechanism-based GAN (shown in Figure 1) to generate RP CT images to overcome the above deficiencies.
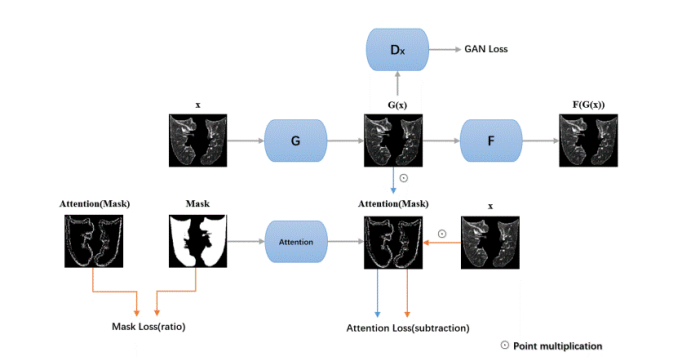
Figure 1. The structure of proposed method. G and F are two generators that generate normal lungs and pneumonic lungs, respectively. Dx is the discriminator to distinguish whether the image is real.
Material and Methods
CT images and dose files were obtained from 300 patients diagnosed with lung cancer who received radical radiotherapy at Tianjin cancer hospital between 2013 to 2019. Of these 62 were diagnosed with RP.
Two experiments were used to prove the utility of our algorithm. For the first experiment, the model was trained by unpaired patients with and without RP. Then the heat map of the differences between the synthetic RP images and original normal lung patient is compared to illustrate that the model can generate realistic RP patterns(Shown in Figure 2).
Second, after randomly splitting the dataset into a training set and a test set at a ratio of 7:3. we trained a DL(RseNet10) model for RP classification on the training set with and without the synthetic images and tested it to show that adding synthetic images can improve DL performance.
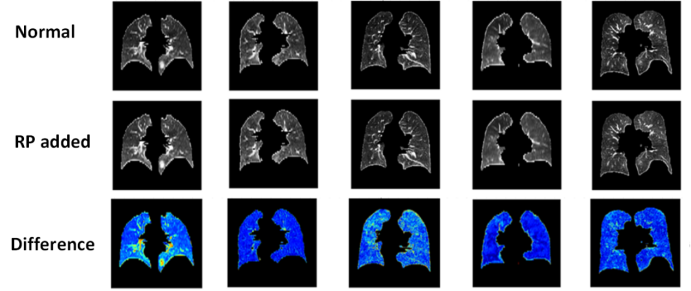
Figure 2. Representative results of our work. Every column is a single patient. The “normal” row is the CT without radiation pneumonitis. In “RP added” row, our GAN-based method has added radiation pneumonitis to the image. The different row contains the difference between these two images to show what the algorithm has changed in the image.
Results
As shown in Figure 2, the synthetic RP images have more complex textures in the lungs compared to normal lungs. As shown in table 1, the performance of the DL model is lower if it is trained only using the original dataset. Synthetic data can improve the model's classification ability, resulting in an improvement in 46% of sensitivity,3% of accuracy and 12% of overall AUC.
Table1 Classification model performance trained on the dataset.
methods | AUC | ACC | SEN | SPE |
Original data
| 0.55(0.52-0.57)
| 0.63(0.60-0.65)
| 0.27(0.26-0.29)
| 0.79(0.77-0.81)
|
Original data + synthetic data
| 0.67(0.66-0.70)
| 0.66(0.64-0.67)
| 0.73(0.71-0.77)
| 0.63(0.60-0.64)
|
Conclusion
Our method can generate realistic RP images and can be utilized as a RP data augmentation method to assist in overcoming the sample imbalance problem and lack of annotated data.