Deep learning-based metal artifact reduction in CT scans for US-based cardiac motion management
Sathyathas Puvanasunthararajah,
Australia
PO-1876
Abstract
Deep learning-based metal artifact reduction in CT scans for US-based cardiac motion management
Authors: Sathyathas Puvanasunthararajah1, Davide Fontanarosa1, Marie-Luise Wille2, Saskia Camps3
1Queensland University of Technology, School of Clinical Sciences, Brisbane, Australia; 2Queensland University of Technology, School of Mechanical, Medical & Process Engineering, Brisbane, Australia; 3EBAMed SA, Research and Development, Geneva, Switzerland
Show Affiliations
Hide Affiliations
Purpose or Objective
Cardiac radioablation has evolved as a promising non-invasive modality for the treatment of cardiac arrhythmias. However, the complexity of cardiac motion may challenge the accuracy of radiation dose delivery. A simultaneous ultrasound (US) and planning computed tomography (CT) acquisition could be a valuable tool to address the limitations of CT in terms of distinguishing between cardiac contraction and respiratory motion and to the artifacts created by cardiac implanted devices. However it can result in US transducer-induced metal artifacts on the planning CT scans. To reduce the impact of these artifacts, a metal artifact reduction algorithm has been developed based on a deep learning Generative Adversarial Network (CycleGAN) and it has been compared with the Metal Deletion Technique (MDT, ReVision Radiology) algorithm.
Material and Methods
The CycleGAN network was trained using clinical CT scans from 70 patients which were extracted from publicly available COVID19-CT-Dataset consisting of 3515 paired CT slices with and without simulated US transducer-induced metal artifacts (Artifact and Reference, respectively). Then, a phantom (ART-211 male) CT scan with real artifacts, and the clinical CT scans with simulated artifacts consisting of 650 CT slices from 14 patients were used to create the artifact-corrected CT scans after MDT and CycleGAN applications. The structural similarity (SSIM) image quality metric and HU value-based root mean square error (RMSE) were calculated between the Reference CT scan and the corresponding Artifact and artifact-corrected CT scans. Higher SSIM values, which range from 0 to 1, indicate better image quality, while RMSE values should be low for better artifact reduction.
Results
In Figure 01 an example of the effect of the application of these algorithms is shown. For the phantom CT scan, the calculated mean SSIM values for the Artifact and the artifact-corrected CT scan after MDT and CycleGAN applications were 0.86, 0.93, and 0.95, respectively. The mean RMSE value for the Artifact CT scan was 63.18; it reduced to 33.17 after MDT, and 31.83 after CycleGAN application. For the clinical CT scans, the mean SSIM values for the Artifact and the artifact-corrected CT scans after MDT and CycleGAN applications were 0.76, 0.91, and 0.92, respectively. The mean RMSE value for the Artifact CT scans was 84.83; it reduced to 33.85 after MDT, and to 30.86 after CycleGAN application.
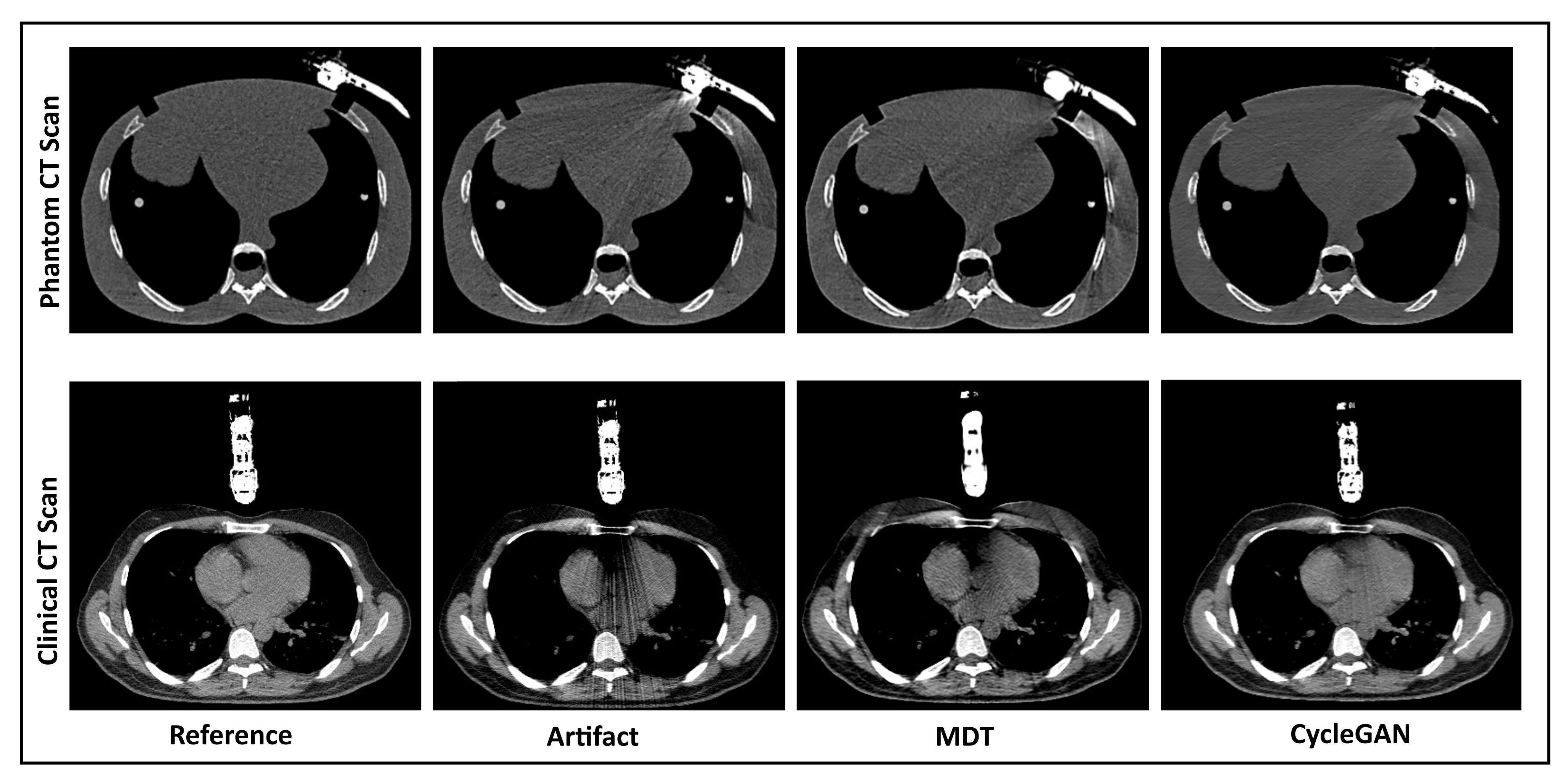
Figure 01: Images from left to right: the CT slice without artifact (Reference), CT slice with artifact (Artifact), and the artifact-corrected CT slices after the application of MDT and CycleGAN algorithms, respectively.
Conclusion
The results of this study show that the application of MAR algorithms reduces the impact of US transducer-induced metal artifacts on planning CT scans. In comparison to iMAR and MDT, the application of developed CycleGAN on planning CT scans performs better in reducing these metal artifacts.