Integrative Radiomic-Clinical Model Improves Long-term Prognostication of High-risk Prostate Cancer
Chi Fung CHING,
China, Hong Kong Special Administrative Region
PO-2126
Abstract
Integrative Radiomic-Clinical Model Improves Long-term Prognostication of High-risk Prostate Cancer
Authors: Chi Fung CHING1, Chi Fung Ching2, Chun Hin Lam1, On Yi Lui1, Chung Ki Kwong1, Yee Hin Lo1, Wang Hin Chan1, Wan Shun Leung1, Shara WY Lee1
1The Hong Kong Polytechnic University, Department of Health Technology and Informatics, Hong Kong, Hong Kong (SAR) China; 2Hong Kong Sanatorium & Hospital, Department of Radiotherapy, Hong Kong, Hong Kong (SAR) China
Show Affiliations
Hide Affiliations
Purpose or Objective
Prostate-only radiotherapy (PORT) has been used for treating high-risk prostate cancer (HRPCa), with the objective of balancing disease control and quality of life. However, over half of these patients suffer from disease progression within 5 years after PORT. Currently, conventional clinical factors are incapable to identify HRPCa patients who may benefit more from PORT than other aggressive treatments. In precision medicine, radiomics facilitates prognostic prediction in many cancers. In this study, we investigated the ability of pre-treatment planning computed tomography (pCT)-based radiomic features combined with clinical factors in predicting 5-year progression-free survival (PFS) of HRPCa patients following PORT.
Material and Methods
Clinical data and pCT of 100 biopsy-confirmed HRPCa patients treated by PORT at the Hong Kong Princess Margaret Hospital were retrospectively analysed. Based on the Transparent Reporting of a Multivariable Prediction Model for Individual Prognosis or Diagnosis guideline, temporal stratification by the PORT start date was performed, with patients divided into the training (n = 75) and independent validation cohort (n = 25). Radiomic (R) features were extracted from gross tumour volume (GTV) on pCT according to the Image Biomarker Standardisation Initiative protocol. Clinical (C) features used for building the C model included prostate specific antigen (PSA), Gleason score (GS), Roach score (RS) and GTV volume. Mann-Whitney U test was used for selecting radiomic features with high clinical relevance for R model building. The radiomic-clinical (RC) model was generated by combining the two models. Ridge regression with 5-fold cross-validation was iterated 100 times in the training cohort of each model, with a model score (i.e. coefficient * feature + intercept) rendered by each model for each individual patient. The classification performance of the three models on 5-year PFS was evaluated in the independent validation cohort using the area under receiver-operating-characteristics curve (AUC), and the Delong’s test for model comparisons.
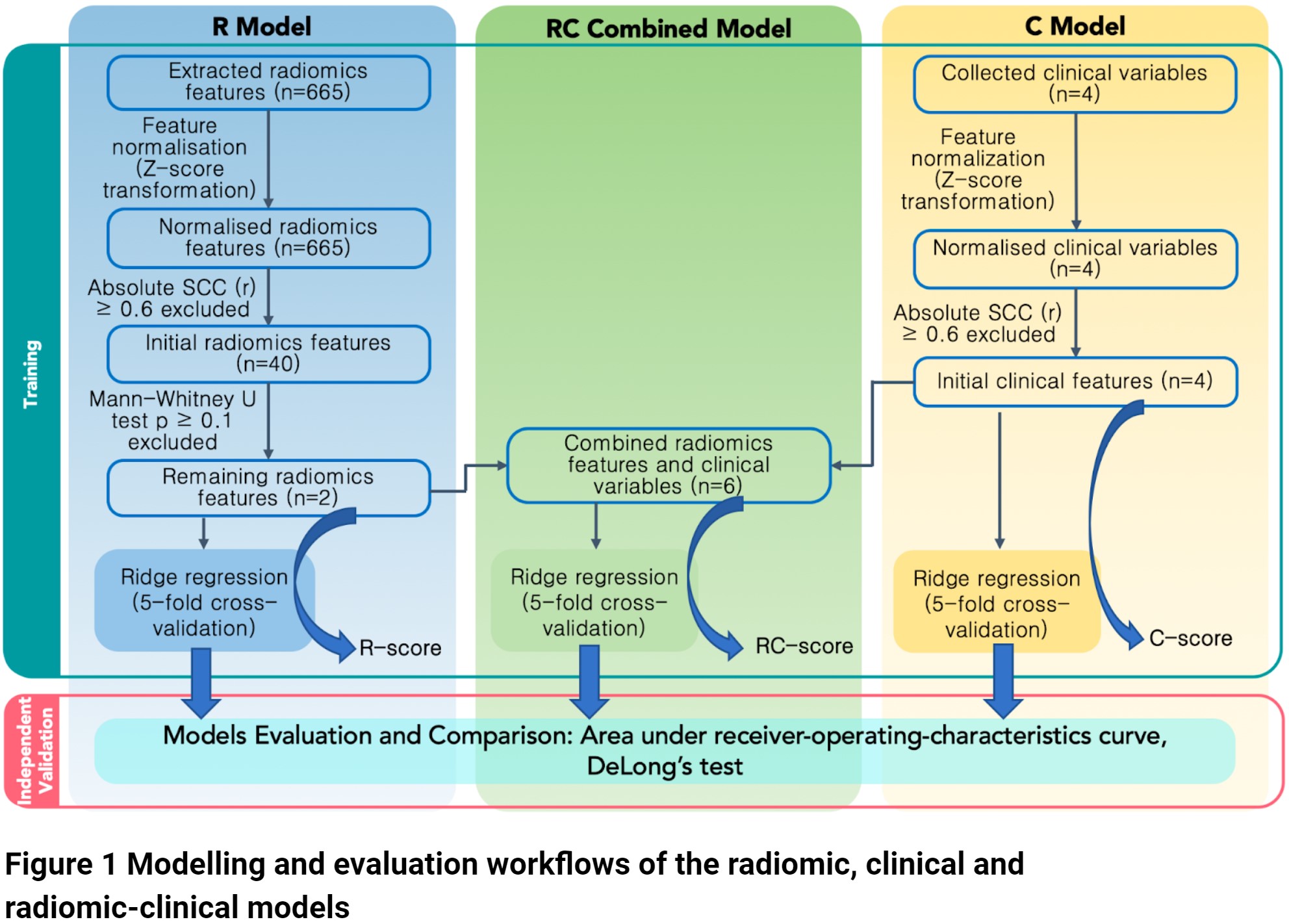
Results
The RC model containing six predictive features (GTV shape flatness, root-mean-square on fine Laplacian of Guassian-filtered image, PSA, GS, RS and GTV volume) has the best performance (AUC = 0.797, 95%CI = 0.768-0.826), which significantly outperformed the R-model (AUC = 0.795, 95%CI = 0.774-0.816) and C-model (AUC = 0.625, 95%CI = 0.585-0.665) in the independent validation cohort. Besides, only the RC model score significantly classified patients in both the training and independent validation cohorts into progression and progression-free groups regarding their 5-year PFS (p < 0.05).
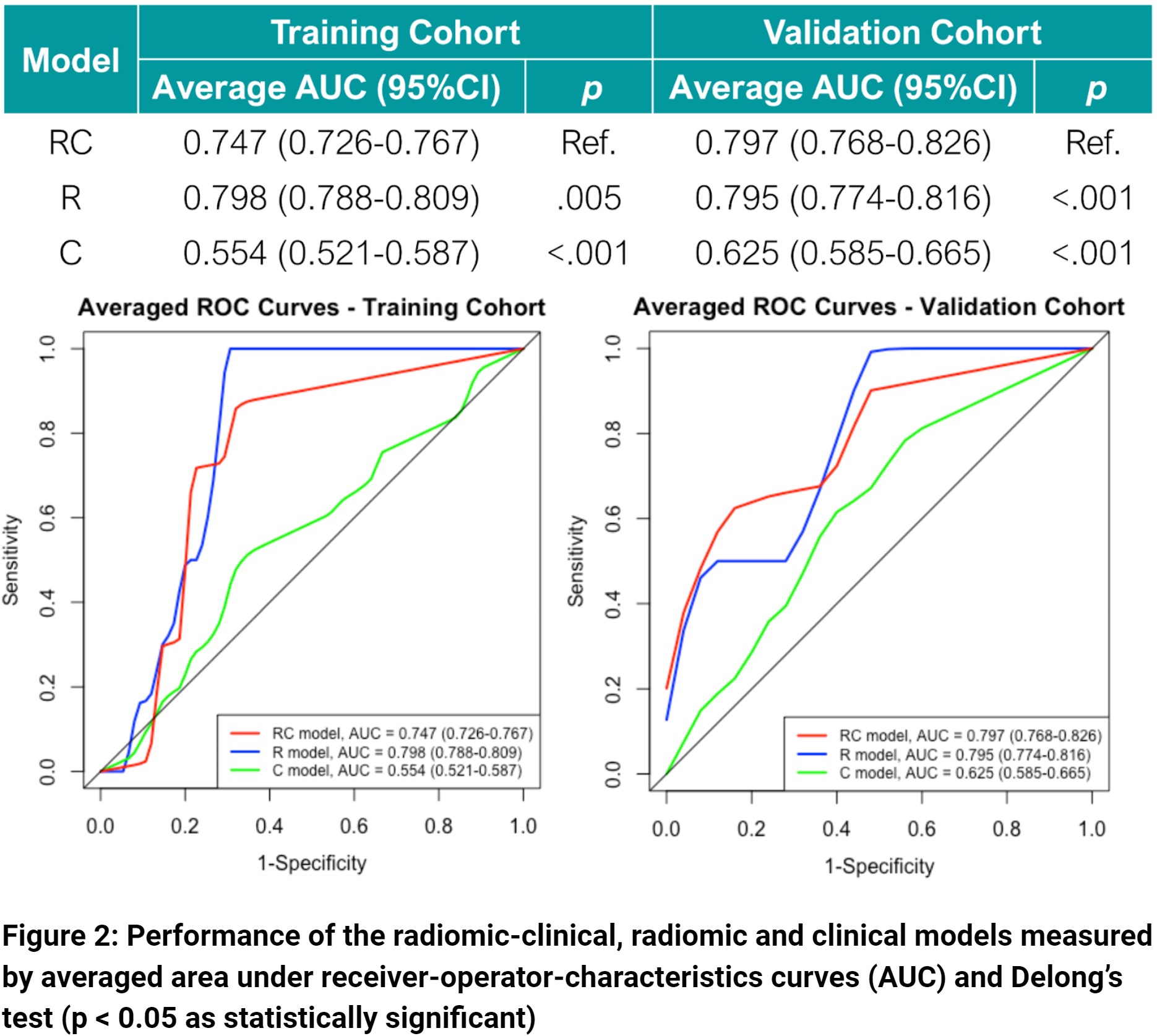
Conclusion
Radiomic features extracted from the pCT adds value to conventional clinical factors in the prognostication of HRPCa in our cohort. Our findings confirm the potential of incorporating radiomics into clinical decision-making in the era of personalised medicine.