Machine learning and image-oriented methods for head and neck cancer treatment outcome prediction
PO-2114
Abstract
Machine learning and image-oriented methods for head and neck cancer treatment outcome prediction
Authors: Bao Ngoc Huynh1, Aurora Rosvoll Groendahl1, Oliver Tomic1, Ingerid Skjei Knudtsen2,3, Frank Hoebers4, Wouter van Elmpt4, Eirik Malinen3,5, Einar Dale6, Cecilia Marie Futsaether1
1Norwegian University of Life Sciences, Faculty of Science and Technology, Ås, Norway; 2Norwegian University of Science and Technology, Department of Circulation and Medical Imaging, Trondheim, Norway; 3Oslo University Hospital, Department of Medical Physics, Oslo, Norway; 4Maastricht University Medical Center, Department of Radiation Oncology (MAASTRO), Maastricht, The Netherlands; 5University of Oslo, Department of Physics, Oslo, Norway; 6Oslo University Hospital, Department of Oncology, Oslo, Norway
Show Affiliations
Hide Affiliations
Purpose or Objective
Different machine learning (ML) methods, including deep learning (DL) with image-oriented methods such as convolutional neural networks (CNN), were used to predict disease free survival (DFS) and overall survival (OS) in two cohorts of head and neck cancer (HNC) patients.
Material and Methods
HNC patients from two centers, Oslo University Hospital (OUS, N=139) and Maastricht University Medical Center (MAASTRO, N=99) with 18F-FDG PET/CT images acquired before radiotherapy, were included. Two types of input data were analyzed: (D1) clinical factors and (D2) PET/CT images with delineated primary tumors (GTVp) and affected lymph nodes (GTVn). The prediction targets DFS and OS were treated as binary responses, in which class 1 indicated an event.
Seven models (M1-M7) with increasing complexity levels were trained and validated on the OUS dataset using nested 5-fold cross-validation. The external MAASTRO dataset was used for testing the models on previously unseen data. Five performance metrics were computed: (I) Accuracy, (II) Area Under the Receiver Operating Characteristic Curve (AUC), (III) Matthews correlation coefficient (MCC), F1 score on class 1 (IV) and class 0 (V) separately. As the event ratios were different between the two datasets (DFS: 49% (OUS), 41% (MAASTRO); OS: 60% (OUS), 54% (MAASTRO)), all metrics were calculated from 1000 bootstrap samples from each dataset, using a 1:1 ratio between the two classes.
Prediction models based on clinical factors only (D1) were constructed using the conventional ML methods logistic regression (M1) and random forest (M2), as well as two DL approaches: one using a simple neural network (M3) and the other using a neural network with interactions between network nodes (M4) to learn possible feature interactions within the data.
A downscaled 3D version of the EfficientNet CNN was used to derive patterns or possibly radiomics features from 3D image input (D2). With this CNN, three outcome prediction models using the following different groups of image input were evaluated: CT and PET (M5); CT, PET and GTVp (M6); CT, PET, GTVp and GTVn (M7).
Results
For DFS (Figure 1), models based on clinical input data (M1-M4) had the overall poorest external validation results due to their considerably low MCC and class 0 F1 scores, reflecting a high number of false positive predictions for the MAASTRO dataset. The CNNs trained on CT and PET images, with and without the GTVp (M5&M6), obtained the highest performance metrics.
For OS (Figure 2), only CNN models (M5-M7) maintained their performance under external validation while other models (M1-M4) overfitted to the OUS dataset as indicated by the decrease in performance for the MAASTRO set. Model M6, trained on CT, PET and GTVp, obtained the best performance in almost all metrics.
Conclusion
CNN models based on CT and PET images without inclusion of clinical data can achieve better and more generalized performance in a multi-center setting when predicting DFS and OS than models based solely on clinical information.
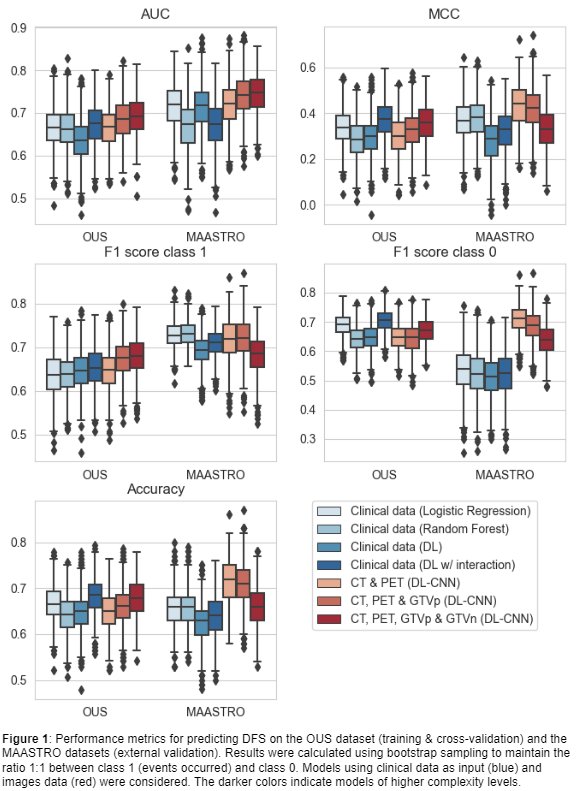
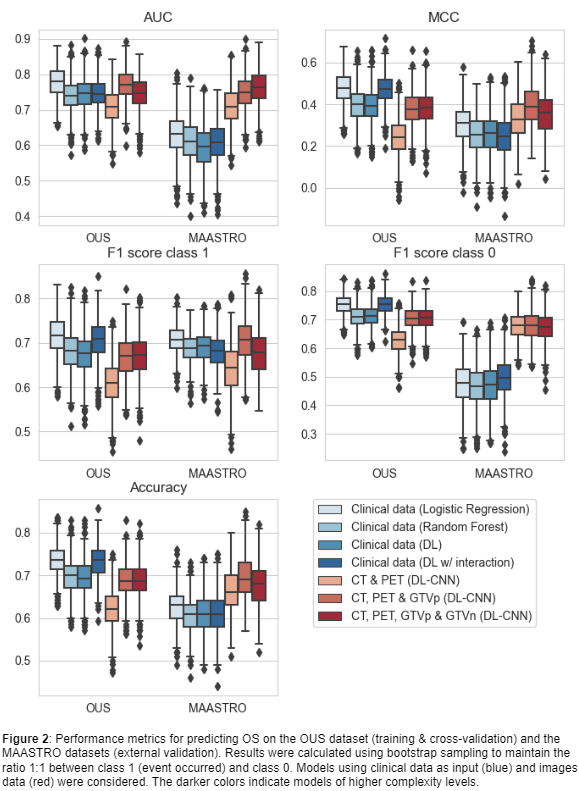