Impact of normalisation methods for longitudinal MR images on radiomic features.
Aaron Rankin,
United Kingdom
PO-2109
Abstract
Impact of normalisation methods for longitudinal MR images on radiomic features.
Authors: Aaron Rankin1, Marianne Aznar1, Angela Davey1, Robin Portner2, Alan McWilliam1
1University of Manchester, Division of Cancer Sciences, Manchester, United Kingdom; 2Lancashire Teaching Hospitals NHS Foundation Trust, Clinical Oncology, Preston, United Kingdom
Show Affiliations
Hide Affiliations
Purpose or Objective
Pre-processing steps for extracting radiomic features from MR images have been established in the literature. However, with the introduction of MR-guided radiotherapy (MRGRT), temporally dense datasets are becoming more available and promise to provide greater detail on biological processes during treatment. It is unclear how well-established pre-processing techniques will map into this new domain. In this work, we compare 7 potential normalisation techniques on longitudinal feature selection in MRGRT and present a novel feature selection methodology for longitudinal data.
Material and Methods
All treatment images of 20 patients with prostate cancer receiving 36 Gy in 5 fractions were collected. All patients underwent 6-months ADT prior to MRGRT on a 1.5T MR-Linac.
Seven image normalisation techniques were implemented:
• Histogram matching (HM) to 1) inter-patient to a selected template image and 2) intra-patient to fraction 1 image and 3) combination, intra-patient to fraction 1 followed by inter-patient to a template patient.
• Intensity rescaling using median signal in 3 ROIs (Fig 1a). Each image was rescaled so the median signal in the ROI was equal to fraction 1. Two out-of-field ROIs were defined in the psoas and glute muscles. Additionally, a region in the prostate was selected.
• Finally, raw images were analysed (i.e. no normalisation).
The entire prostate was contoured by an expert clinician and a small volume in the glute and psoas muscles was defined by a PhD student with clinical guidance. In total, 87 features (FTs) were extracted using pyRadiomics. FTs at fraction 1 were correlated with volume and were removed if Spearman correlation > 0.6.
Next, redundant FTs (i.e. correlated with another FT) were eliminated using cross-correlation. For each patient, longitudinal-FT pair correlations were calculated and those with correlation > 0.6 were removed. The remaining FTs were ranked for each normalisation across all patients and the 5 most common FTs for each normalisation were then selected.
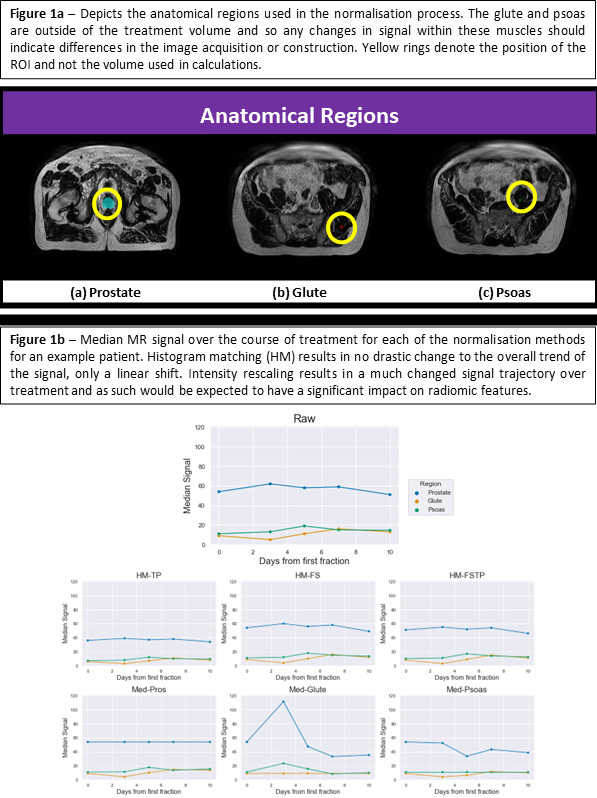
Results
Fig 1b shows the impact of normalisation on the signal trajectory of each ROI. Normalisation has little effect on the number of volume-dependence FTs (Table 1), or the median number of remaining FTs per patient. However, the choice of normalisation impacts which FTs are selected (Table 1).
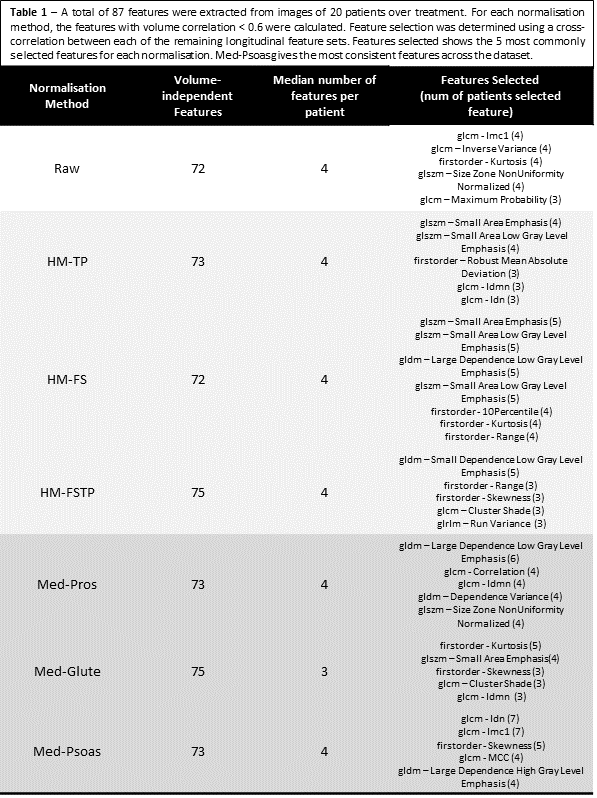
Conclusion
We have shown that established normalisation methods can suppress or enhance subtle changes in radiomic FTs across treatment: this suggests that methods developed on single timepoints may not be suitable for longitudinal data. Despite large changes in trajectories, normalisation has little impact on the number of FTs remaining after application of a FT selection pipeline tailored to longitudinal data. We preliminary recommend using the Psoas muscle for normalisation as it produced the most consistent FT selection across patients. Further work is needed to investigate the impact this has on predictive capabilities of radiomic models.