Impact of breathing and image filtering on radiomic features derived from 4D-CT in early-STAGE NSCLC
PO-2108
Abstract
Impact of breathing and image filtering on radiomic features derived from 4D-CT in early-STAGE NSCLC
Authors: Stefania Volpe1, Federico Mastroleo2, Aurora Gaeta3, Mattia Zaffaroni1, Matteo Pepa1, Maria Giulia Vincini1, Sara Raimondi3, Lars Johannes Isaksson1, Cristiano Rampinelli4, Marta Cremonesi5, Sara Gandini3, Matthias Guckenberger6, Roberto Orecchia7, Barbara Alicja Jereczek-Fossa1
1Istituto Europeo di Oncologia IRCCS, Department of Radiation Oncology, Milan, Italy; 2Università del Piemonte Orientale, Department of Translational Medicine, Novara, Italy; 3Istituto Europeo di Oncologia IRCCS, Department of Experimental Oncology, Milan, Italy; 4Istituto Europeo di Oncologia IRCCS, Department of Radiology, Milan, Italy; 5Istituto Europeo di Oncologia IRCCS, Radiation Research Unit, Milan, Italy; 6University Hospital Zurich, Department of Radiation Oncology, Zurich, Switzerland; 7Istituto Europeo di Oncologia IRCCS, Scientific Directorate, Milan, Italy
Show Affiliations
Hide Affiliations
Purpose or Objective
While 4D-computed tomography (CT) simulation represents a gold standard in stereotactic body radiotherapy (SBRT) for early-stage non-small cell lung cancer (ES-NSCLC), dedicated investigations on these images in radiomic studies are limited. This work aims to test the coefficient of variation (COV) of radiomic features across 10 respiratory phases and after applying different filtering methods.
Material and Methods
Seventy 4D-CTs acquired with the same scanner and acquisition parameters at a single Institution were retrieved. Pre-processing and features extraction were implemented using Pyradiomics v3.0.1. Features were subdivided into 7 classes, namely first order, gray level co-occurrence matrix, gray level dependence matrix, gray level run length matrix, gray level zone matrix, neighboring gray tone difference matrix and shape. Null features in more than 90% of the cases in all respiratory phases were excluded. For each feature, the COV between the ten phases measurements was calculated for each patient [COV = (standard deviation/average) × 100]. Each feature is then represented by the average COVs among patients. The average COV was then classified as ≤5%, 5%20%.
Results
Almost 2000 features, mainly classified within the gray level co-occurrence matrix category, were extracted. Considering pre-processing methods, the majority of features derived from the wavelet (all permutations), lpb-3D and log-sigma filters (n= 744, 279 and 279, respectively). Qualitatively, COVs> 20% were observed across all categories and filters. Specifically, COVs>20% were more the most frequent in the gray level zone matrix and in the neighboring gray tone difference matrix categories. The application of pre-processing determined a different distribution of COVs, with a predominance of stable features (COVs≤5%) in the lpb-2D and lpb-3D methods, while the largest variability was observed when the logarithm and log-sigma filters were used (Fig1).
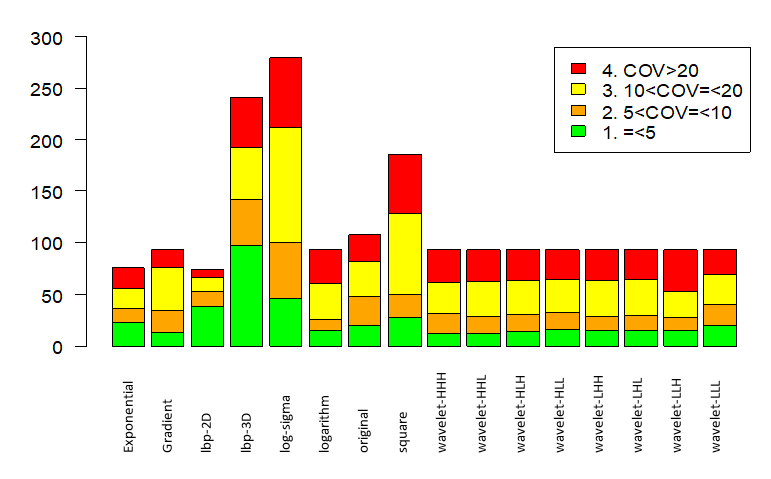
Conclusion
Radiomic features show a significant range of variability across respiratory phases. In addition, as our results suggest, not only breathing but also the application of specific filtering techniques can affect features stability. While the impact of COVs in clinical prognostic modelling is being assessed by our group, we can affirm that these preliminary results have shed a light on the potentials of implementing 4D-based analysis in radiomic studies for ES-NSCLC.