Demonstrating variability in radiomic analysis due to inconsistent conversion from contour to mask.
Emiliano Spezi,
United Kingdom
PO-2103
Abstract
Demonstrating variability in radiomic analysis due to inconsistent conversion from contour to mask.
Authors: Philip Whybra1, Emiliano Spezi1
1Cardiff University, School of Engineering, Cardiff, United Kingdom
Show Affiliations
Hide Affiliations
Purpose or Objective
Standardised and repeatable radiomics is needed for clinical use. Traditional radiomics is guided by a segmentation, and it is well known that inter- and intra- user contour variation influences features. The international standard to transmit, store, and display medical imaging and radiotherapy information (DICOM) saves a contour as coordinate points which must be converted to a binary mask for radiomics analysis. In a prominent standardisation effort using consensus benchmarking [1], no one method was chosen for mask conversion. We demonstrate slight differences in mask conversion of the same data during import can, alone, affect patient clustering and subsequent modelling.
Material and Methods
We used multimodal imaging (CT, MRI, PET) from a public soft-tissue sarcoma (STS) dataset of 51 patients [1], available in DICOM and NIfTI formats. We interfaced our radiomics software developed in-house with selected commercial and research-based medical imaging software APIs (MIM, CERR, and MICE Toolkit). Our radiomics pipeline is compliant with standardised radiomics benchmarks [1]. We extracted 158 features from each region.
We simulated a mixed institution radiomics collection using different software to import the DICOM data, and then the same benchmarked algorithms to extract the features. We compared results to a baseline extraction using the NIfTI files, which already store contours as masks. For the mixed-import dataset, the 51 patients were divided into 4 group and then recombined after feature extraction. The DICOM data was imported with 1) CERR, 2) MIM, 3) MICE Toolkit, and 4) MICE Toolkit (with mask conversion using super-sampling). The mixed and baseline features were normalised (z-score) separately and hierarchical clustering of patients compared (with R packages cluster and dendextend), using a dendrogram entanglement (E) measure [2].
Results
Small mask discrepancies were measured between imports of the DICOM data with different software. A visual representation of typical mask discrepancy is show in Fig. 1. These mask differences resulted in small variations in raw feature values which, with z-score normalisation, affected clustering. This is shown in dendrogram comparisons in Fig 2. Mask discrepancy was present in the MRI and PET imaging and had a greater effect on resulting cluster differences (E: CT=0, MRI=0.26, PET=0.22).
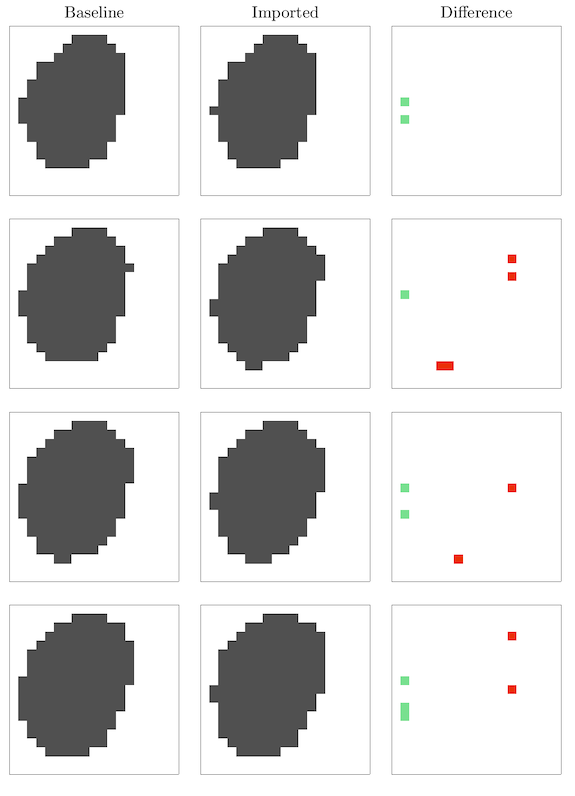
Fig.1. Mask discrepancy example.
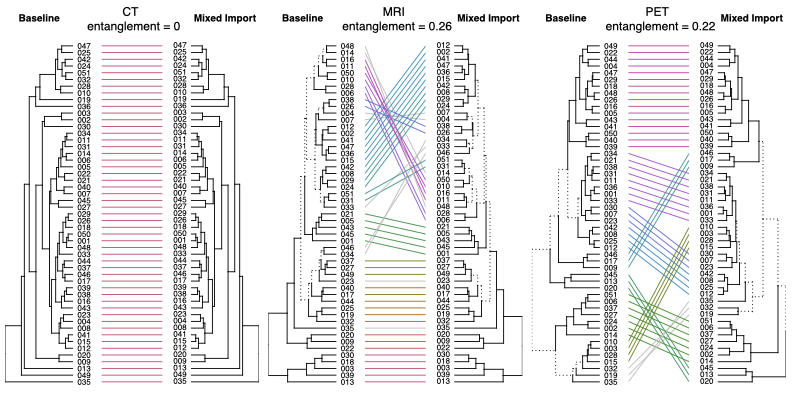
Fig.2 Mask discrepancies culminate in feature differences that cause cluster change.
Conclusion
We find radiomic analysis of the same data diverged due to inconsistent mask conversion between software. This work is relevant for multicentre and federated learning studies that access data from different institutions where use of the same mask conversion is not guaranteed. To mitigate this issue, one can incorporate mask perturbations [3] to assess feature susceptibility to mask variation and to ensure only robust features are used.
[1] 10.1148/radiol.2020191145
[2] 10.1093/bioinformatics/btv428
[3] 10.1038/s41598-018-36938-4