CT-based radiomics for outcome prediction in oropharyngeal cancer patients treated with curative RT
PO-2102
Abstract
CT-based radiomics for outcome prediction in oropharyngeal cancer patients treated with curative RT
Authors: Stefania Volpe1, Aurora Gaeta2, Francesca Colombo1, Mattia Zaffaroni1, Maria Giulia Vincini1, Matteo Pepa1, Lars Johannes Isaksson1, Irene Turturici1, Alessia Casbarra3, Giulia Marvaso1, Anna Maria Ferrari1, Giulio Cammarata2, Riccardo Santamaria1, Sara Raimondi2, Francesca Botta4, Mohssen Ansarin5, Marta Cremonesi6, Roberto Orecchia7, Daniela Alterio1, Barbara Alicja Jereczek-Fossa1
1Istituto Europeo di Oncologia IRCCS, Department of Radiation Oncology, Milan, Italy; 2Istituto Europeo di Oncologia IRCCS, Department of Experimental Oncology, Milan, Italy; 3Istituto Europeo di Oncologia IRCCS, Department of Radiation Oncolgy, Milan, Italy; 4Istituto Europeo di Oncologia IRCCS, Department of Medical Physics, Milan, Italy; 5Istituto Europeo di Oncologia IRCCS, Division of Otolaryngology and Head and Neck Surgery, Milan, Italy; 6Istituto Europeo di Oncologia IRCCS, Radiation Research Unit, Milan, Italy; 7Istituto Europeo di Oncologia IRCCS, Scientific Directorate, Milan, Italy
Show Affiliations
Hide Affiliations
Purpose or Objective
To assess whether CT-based radiomics could improve the prediction of overall survival (OS) and local progression free survival (LPFS) in patients with oropharyngeal cancer (OPC) treated with curative-intent IMRT.
Material and Methods
Consecutive OPC patients with radiologically detectable primary tumor treated between 2005 and 2021 were included. Analyzed clinical variables included gender, age, smoking history, staging, subsite, HPV status and blood counts (e.g. baseline hemoglobin, platelets, neutrophil/lymphocyte ratio and lymphocyte/monocyte ratio). Radiomic features were extracted from manually-segmented GTVs of the primary tumor using Pyradiomics. For the analysis, univariate proportional hazard and penalized Cox regression models were applied to clinical and radiomic features, respectively; a radiomic score (RS) was obtained to stratify patients. Lasso regression was applied to select potentially significant radiomic features based on the Spearman correlation coefficient. A 10-fold cross-validation with 500 bootstrapping repetitions was performed to minimize bias and overfitting. As many as 3 and 5 features were used for OS and LPFS prediction, respectively. Three models, namely clinical, radiomic and clinical-radiomic models were built including, respectively, clinical variables only, radiomic variables only and both. For each model, C-index and respective CIs were obtained from the bootstrap estimate. The likelihood-ratio test was used to compare the performances of the models, with a p-value<0.05 indicating that radiomics led to an improvement in predictivity.
Results
One-hundred five patients, predominately male, were included in the analysis. Median age was 59 (IQR: 52-66) years, and stage IVA was the most represented (70%). HPV status was positive in 63, negative in 7 and missing in 35 patients. Median follow-up was 6.3 (IQR: 5.5-7.9) years. The calculation of the RS enabled to successfully stratify patients according to both OS (p<0.0001) and LPFS (0.0002) (Fig1). The model including both clinical and radiomic variables outperformed the other two, with a C-index of 0.82 [CI: 0.80-0.84] for OS and 0.86 [CI: 0.86-0.89] for LPFS.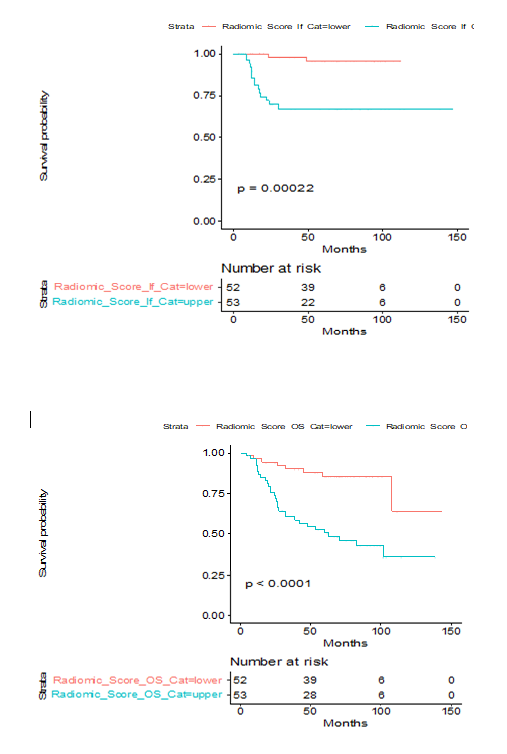
Conclusion
Our results show that radiomics could bring clinically significant informative content in this scenario. The best performances were obtained by combining clinical and quantitative imaging variables, thus suggesting the potentials of complex modeling for outcome predictions in this setting of patients.