Machine learning prediction of Dice similarity coefficient for accuracy evaluation
PO-2096
Abstract
Machine learning prediction of Dice similarity coefficient for accuracy evaluation
Authors: Yun Ming Wong1, Ping Lin Yeap2, Ashley Li Kuan Ong2, Hong Qi Tan2, Wen Siang Lew1, James Cheow Lei Lee1,2
1Nanyang Technological University, School of Physical and Mathematical Sciences, Singapore, Singapore; 2National Cancer Centre Singapore, Division of Radiation Oncology, Singapore, Singapore
Show Affiliations
Hide Affiliations
Purpose or Objective
Following the advent of highly conformal radiotherapy techniques, patient anatomic variations are having a greater impact on the daily dose distributions. This calls for regular adjustment of treatment plan, a process known as adaptive radiotherapy (ART). Deformable image registration (DIR), a technique to transform one image to another, is indispensable in an ART workflow. While contour-based metrics, e.g. Dice similarity coefficient (DSC), are commonly used for DIR accuracy evaluation, they require the manual delineation of contours, which is a bottleneck in the radiotherapy workflow due to its laborious nature. In this work, we presented a novel method of predicting DSC using DVF-based metrics by applying several machine learning models, to achieve a quick DIR validation process without much human intervention.
Material and Methods
Our study involved data from 20 low-risk prostate cancer patients. For each patient, the planning CT (pCT) image and fractional cone-beam CT (CBCT) images were imported into RayStation 10A (RaySearch Laboratories, Stockholm, Sweden), along with the manual contours delineated on the images. DIR was then performed using the pCT image as the reference image and the fractional CBCT images as the target images. Various DVF-based metrics, such as the minimum and maximum DVF magnitude, as well as the DSC which measures the overlap between the deformed pCT contours and the manual CBCT contours were obtained from RayStation. Using the extracted DVF-based metrics as features, machine learning was done to predict DSC. Analysis was done on four sets of data, i.e. 1) prostate only, 2) bladder only, 3) rectum only and 4) all the organs combined. The first three sets have the same total number of examples (761) while the last set has three times as many (2283). Three different models, linear regression (LR), Nu Support Vector Regression (NuSVR) and Random Forest Regressor (RFR) were tested. To achieve the best performance for NuSVR and RFR, the hyperparameters were optimised using MAE through 10-fold validation. The models with the optimal hyperparameters were then used to predict the test set. To evaluate the model performance, 10-fold validation was applied and the average of the mean absolute error (MAE) were computed. As LR did not involve hyperparameter tuning, the inner loop was absent in its training pipeline. Similar to NuSVR and RFR, 10-fold cross validation was used for the model evaluation of LR.
Results
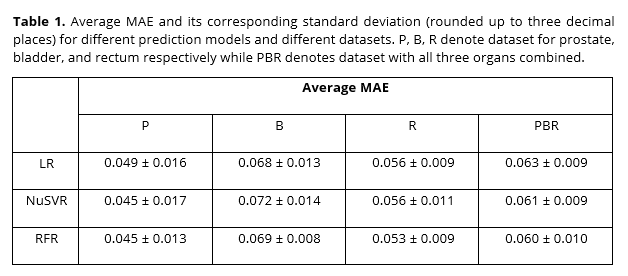
The average MAE with their standard deviation were tabulated in Table 1, for all three models and all four datasets. Overall, RFR showed the best performance, while LR and NuSVR had similar performances. The lowest average MAE achieved was 0.045 while the highest was 0.072.
Conclusion
This study demonstrated the potential of several machine learning models in predicting DSC using DVF-based metrics. For a reliable clinical translation, further analysis on the robustness of these models to uncertainties could be done through quantification of prediction interval.