Toxicity modelling in RT using Bayesian network topology optimization with simulated annealing
PO-2089
Abstract
Toxicity modelling in RT using Bayesian network topology optimization with simulated annealing
Authors: Kailyn Stenhouse1, Philip McGeachy2, Sofia Spampinato3, Kari Tanderup3, Kevin Martell4, Sarah Quirk5, Kathrin Kirchheiner6, Maximilian Schmid6, Michael Roumeliotis7
1University of Calgary, Department of Physics and Astronomy, Calgary, Canada; 2Tom Baker Cancer Centre, Department of Medical Physics, Calgary, Canada; 3Aarhus University Hospital, Danish Center for Particle Therapy, Aarhus, Denmark; 4Tom Baker Cancer Centre, Department of Radiation Oncology, Calgary, Canada; 5Brigham and Women's Hospital, Department of Radiation Oncology, Boston, USA; 6Medical University of Vienna, Department of Radiation Oncology - Comprehensive Cancer Centre, Vienna, Austria; 7Johns Hopkins University, Department of Radiation Oncology and Molecular Radiation Sciences, Baltimore, USA
Show Affiliations
Hide Affiliations
Purpose or Objective
To develop a simulated annealing optimization algorithm for building Bayesian Network (BN) topologies for late toxicity prediction in the EMBRACE I trial on locally advanced cervical cancer after radiochemotherapy.
Material and Methods
EMBRACE morbidity outcomes were used to develop an algorithm for building two separate BN topologies for grade ≥2 cystitis and proctitis, as assessed by CTCAEv3. Baseline and at least one follow-up assessment were required. 40 clinical and treatment features were included as potential network nodes in the optimization. All features were initially included to ensure a direct comparison between the different model-building techniques. Restrictions on node connectivity were specified by experts to ensure that connections followed a logical sequential order (i.e. dosimetric features from treatment cannot be parent nodes of diagnostic features). Topology optimization followed a simulated annealing framework, with the cost function containing terms prioritizing model performance, mutual information between nodes, and simplicity. Results of the simulated annealing optimizations were compared to out-of-box optimization algorithms from the PyAgrum package (Tree-Augmented Naïve Bayes (TAN), Greedy Hill Climbing (GHC), Chow-Liu Optimization (CLO)).
Results
The proctitis model was built using 1108 patients and the cystitis model was built with 1073, excluding patients with bladder wall infiltration. Simulated annealing optimization for both toxicities terminated with convergence following 1000 unchanged iterations. Results for model performance and topology characteristics are outlined in Table 1 and networks are shown in Figure 1.
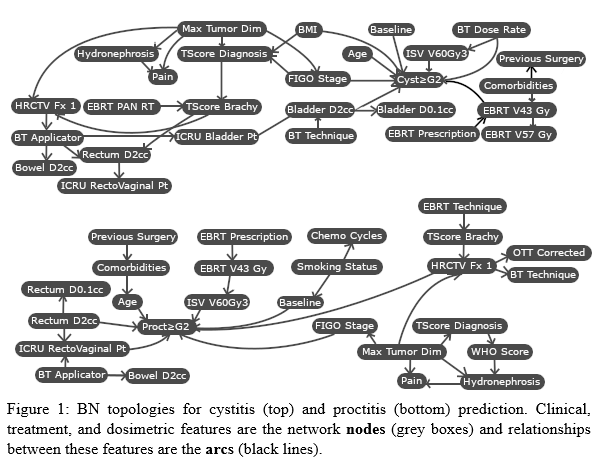
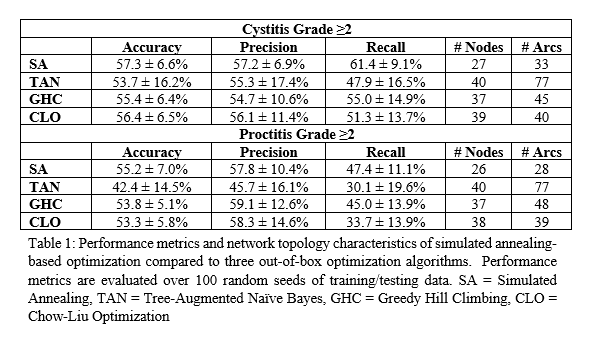
Simulated annealing resulted in models with comparable predictive accuracy to out-of-box optimization techniques (Cystitis: SA=57.3±6.6%, TAN=53.7±16.2%, GHC=55.4±6.4%, CLO=56.4±6.5%. Proctitis: SA=55.2±7.0%, TAN=42.4±14.5%, GHC=53.8±5.1%, CLO=53.3±5.8%). The benefit of the simulated annealing technique is the highly tunable nature of the optimization, allowing for a customizable cost function and expert-specified restrictions placed on connections reducing the of the solution space. As a result, the simulated annealing framework notably resulted in a less complex network than the TAN technique and did not suffer from illogical connections that other models encountered.
Conclusion
Simulated annealing-based optimization resulted in BN topologies with comparable predictive accuracies to other out-of-box optimization techniques. The simulated annealing approach constructed more interpretable and logical topologies while allowing for customization within the cost function and initialization settings to allow for the inclusion of clinical knowledge in the optimization process. Although model accuracy is currently limited, this technique provides a preliminary approach for late toxicity prediction, which remains a complex task with no standard methodology for identifying at-risk patients.