Radiomics and Dosiomics Analysis to predict Pneumonitis after Stereotactic Radioimmunotherapy
Kim Melanie Kraus,
Germany
PO-1327
Abstract
Radiomics and Dosiomics Analysis to predict Pneumonitis after Stereotactic Radioimmunotherapy
Authors: Kim Melanie Kraus1,3,4, Maksym Oresheko2, Denise Bernhardt2,4, Stephanie Elisabeth Combs2,4,5, Jan Caspar Peeken2,5,4, Maksym Oreshko6
1Technical Universty of Munich (TUM), Radiation Oncology, Munich, Germany; 2Technical University of Munich (TUM), Radiation Oncology, Munich, Germany; 3Helmholtz Zentrum München (HMGU) GmbH, German Research Center for Environmental Health, Institute of Radiation Medicine (IRM), Neuherberg, Germany; 4German Cancer Consortium (DKTK), Partner Site Munich and German Cancer Research Center (DKFZ), Munich, Germany; 5Helmholtz Zentrum München (HMGU) GmbH, German Research Center for Environmental Health, Institute of Radiation Medicine (IRM), Munich, Germany; 6LMU Munich, Medical Faculty, University hospital, Munich, Germany
Show Affiliations
Hide Affiliations
Purpose or Objective
The impact of immune checkpoint inhibition (ICI) on the occurrence of post-therapy pneumonitis (PTP) is unclear. We aim to find predictors for the occurrence of pneumonitis after lung stereotactic body radiation therapy using radiomics and dosiomics analysis of 3 D dose distributions for lung cancer and to evaluate the potential impact of ICI on the risk for PTP after combined radioimmunotherapy.
Material and Methods
110 cases of primary lung cancer or pulmonary metastases that received SBRT between 2010 and 2021 were retrospectively collected. Eleven patients received radioimmunotherapy with ICI. In total, 24 suffered from PTP. Clinical data including the occurrence of pneumonitis > grade 0 according to the Common Terminology Criteria for Adverse Events version 5.0 and the use of ICI within an interval of 50 days around SBRT were extracted from patient files. Planning CTs, 3D dose distributions, lung and treatment volume segmentations were selected from the radiotherapy treatment planning system. 104 radiomics and dosiomics features were extracted from the planning CT and 3D dose distributions from each volume of interest (total/ipsilateral lung – GTV, PTV+2cm) using the open-source library Pyradiomics in python, respectively. Feature reduction was performed using 1000-fold bootstrapping using pearson intercorrelation coefficient and the Boruta algorithm. We studied three single predictive models (radiomics, dosiomics, clinical data) and one combined model (dosiomics + radiomics). Different machine learning models including random forest (rf), logistic elastic net regression (glmnet), support vector machine (svmRadial) and logitBoost were trained and tested using a 5-fold nested cross validation approach and Synthetic Minority Oversampling Technique resampling in R [1]. Model performance was analyzed using the area under the receiver operating characteristic curve (AUC) on the test sets of the outer folds.
Results
The best radiomics and dosiomics models (rf, each) predicted PTP better than random (AUC = 0.73 (95 % confidence interval 0.72 – 0.74) and AUC = 0.70 (0.68 – 0.71), respectively). The combination of dosiomics and radiomics features showed the highest predictive performance using random forest (rf) classifier (AUC = 0.79, 0.78 – 0.80). The clinical model did not predict PTP better than random. The AUC values for all models and classifiers applied are depicted in table 1. There was no influence of ICI therapy with an AUC ranging from 0.45 to 0.5 depending on the classifier used.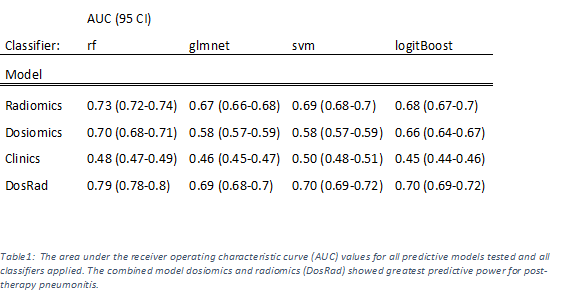
Conclusion
Our results suggest that additional ICI therapy has no impact on the prediction PTP after lung SBRT. Combined dosiomics and radiomics features can improve prediction of PTP after lung SBRT.
References
[1] T.M. Deist, F.J.W.M. Dankers et al., “Machine learning algorithms for outcome prediction in (chemo)radiotherapy: an empirical comparison of classifiers”, Med. Phys. May 2018. doi:10.1002/mp.12967