Uncertainty-aware MR-base CT synthesis for robust proton planning of skull-based tumour
MO-0224
Abstract
Uncertainty-aware MR-base CT synthesis for robust proton planning of skull-based tumour
Authors: Xia Li1,2, Renato Belloti1,3, Gabriel Meier1, Damien Weber4,5,6, Antony Lomax1,3, Joachim Buhmann2, Ye Zhang1
1Paul Scherrere Institut, Center for Proton Therapy, Villigen, Switzerland; 2ETH Zurich, Department of Computer Science, Zurich, Switzerland; 3ETH Zurich, Department of Physics, Zurich, Switzerland; 4Paul Scherrer Institut, Center for Proton Therapy, Villigen, Switzerland; 5University Hospital of Zurich, Department of Radiation Oncology, Zurich, Switzerland; 6Inselspital, Bern University Hospital, University of Bern, Department of Radiation Oncology, Bern, Switzerland
Show Affiliations
Hide Affiliations
Purpose or Objective
Although deep learning-based methods have shown good performance for MR-based CT synthesis (Spadea et al. 2021 Med Phys 48(11)), the associated prediction uncertainty has not yet been estimated conventionally, limiting its clinical application in proton therapy. Therefore, we developed an uncertainty-aware deep learning framework and comprehensively assessed its clinical usefulness for robust proton planning.
Material and Methods
Based on 64 skull-based tumour patients with paired CT-MR images, a conditional generative adversarial network (cGAN) was trained to generate synthetic CT (sCT) by concurrently considering three orthogonal planes of the combined T1 and T2 MR images. Instead of only predicting single values of each sCT voxel, a Bayesian Neural layer was integrated into the cGAN to predict parameters (𝛍, b) of per-voxel Laplacian distributions, from which a series of sCTs was sampled for robust analysis (Figure 1 j). The robust proton plan was optimised by considering three sCTs: one with the per-voxel predicted mean values (𝛍), and the other two with per-voxel predicted mean values plus/minus per-voxel predicted deviations (b). The latter two form the envelope of high-possibility value ranges. The dosimetric differences (dPlan) between the plan derived from sCT (sPlan) and the re-calculated plan (rPlan) on real CT (rCT) were quantified for each patient. The prediction uncertainty-conditioned robust plan was systematically compared to corresponding scenarios of conventional robust planning (global ±3%) and non-robust planning.
Results
From the eight-fold cross-validation, the image differences between sCT and rCT, in terms of the average Mean-Absolute-Error (MAE), are 80.29±4.49 HU for body area, 34.65±1.57 HU for soft tissues and 222.16±13.48 HU for bones. The Dice scores of bones are 90.34±1.15 %. Moreover, the predicted uncertainty distribution positively correlates with the absolute prediction error with the Correlation Coefficient of 0.62±0.01 (Figure 1 c&f). With the uncertainty-conditioned robust optimisation, the rPlans showed much higher agreement with the sPlans (Figure 1 g&h). For example, the absolute difference of D95 is only 1.10±1.24 % of the CTV, in contrast to 1.64±2.71 % and 2.08±2.96 % of the conventional robust optimisation and non-robust optimisation, respectively. This trend is statistically consistent in all indexes of both target and organs-at-risk (Figure 2).
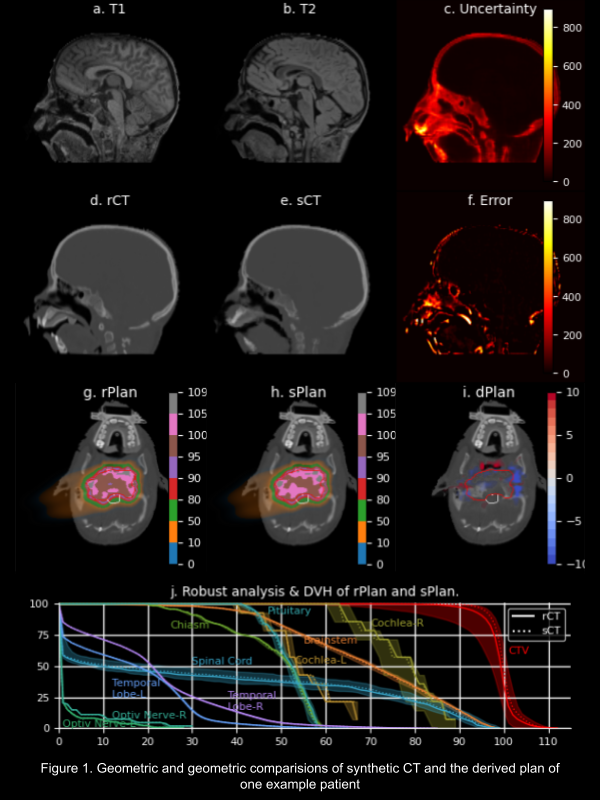
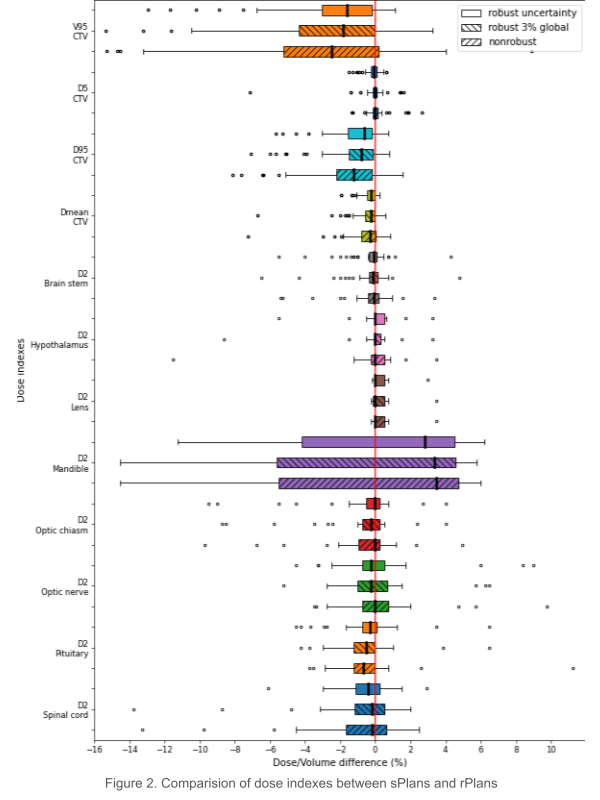
Conclusion
We have demonstrated the high-quality sCT generation from MR images using a modified cGAN-based framework. Besides the clinically acceptable accuracy for proton therapy planning, the associated three-dimensional uncertainty distribution enables the conditioned robust optimisation for improving the plan robustness against prediction error for skull-based tumour treatments. We believe the direct visualisation of the network prediction uncertainty and the subsequential robust analyses are powerful tools for determining the clinical usefulness of synthetic CTs for individual patients.