Predicting survival outcomes for patients with colorectal cancer by explainable pathology predictors
Shenlun Chen,
The Netherlands
MO-0057
Abstract
Predicting survival outcomes for patients with colorectal cancer by explainable pathology predictors
Authors: Shenlun Chen1, Leonard Wee1, Andre Dekker1, Jiazhou Wang2
1Maastricht University Medical Centre+, Department of Radiation Oncology (Maastro), GROW School for Oncology and Reproduction, Maastricht, The Netherlands; 2Fudan University Shanghai Cancer Centre, Department of Radiotherapy, Shanghai, China
Show Affiliations
Hide Affiliations
Purpose or Objective
Although whole slide images (WSI) are the gold standard for tumor grading and postoperative radiotherapy for patients with colorectal cancer (CRC), traditional predictors are usually subjective and machine learning predictors are always lacking interpretability. We proposed an approach to extract explainable and robust predictors from WSIs.
Material and Methods
Total 1155 WSIs from 606 patients in The Cancer Genome Atlas (TCGA) – colon adenocarcinoma (COAD) and rectum adenocarcinoma (READ) public dataset and 108 WSIs from 106 patients in local dataset were enrolled as discovery dataset and external validation dataset, respectively. The visual characteristics of tumor gland formation (G), stroma (S), necrosis (N) and tumor-infiltrating lymphocytes (TILs, L) were investigated as prognostic features, which were predicted by deep learning classifiers. The global average or statistic values (minimal, median and maximum) of WSI’s sub-regions were calculated as candidate explainable pathology features (EPFs). The process of EPFs extraction can be seen in Fig.1. Labels of EPFs followed the format of [data source][sub-region level]_[statistic type], for example, the EPF of G4_min referred to the minimal tumor gland formation characteristics at sub-region level of 4. Then the LASSO-embedded features selection was performed. Finally, we developed the Cox regression model on selected EPFs for overall survival evaluation and projected the EPFs on original WSIs.
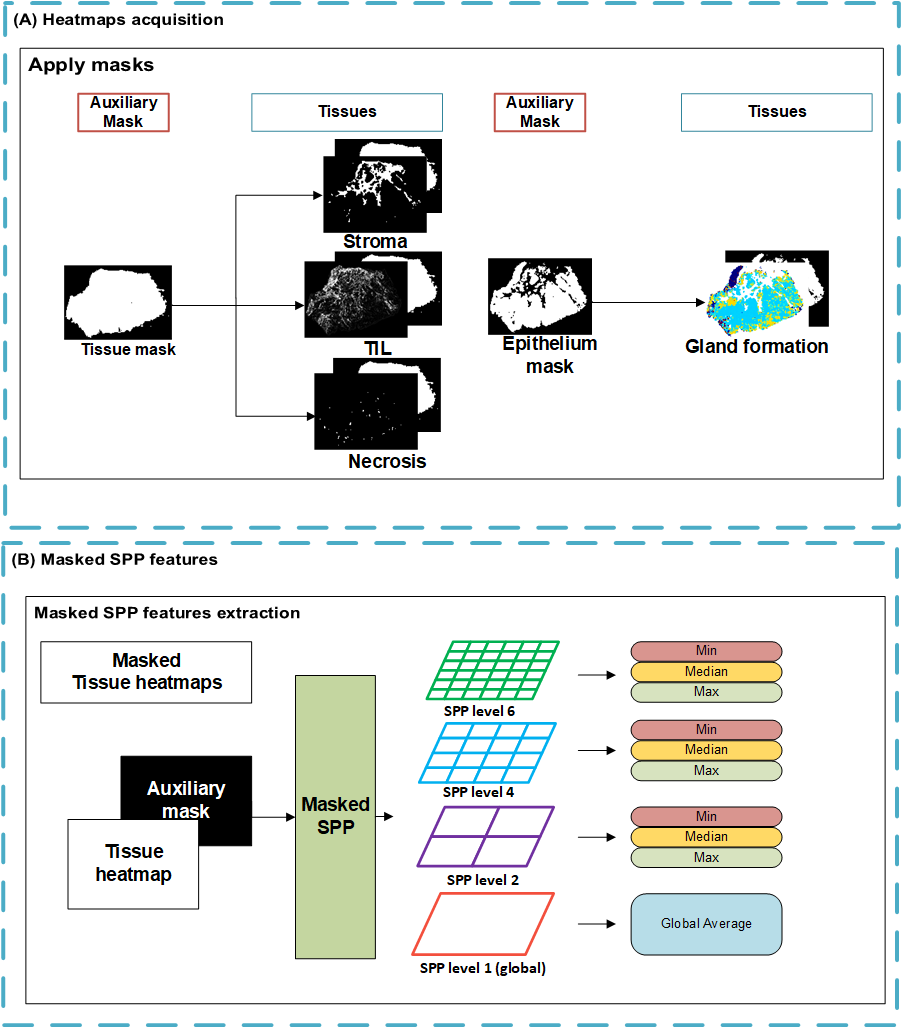
Fig.1 The workflow of EPFs extraction. (A) Four visual characteristics of S, L, N and G as well as auxiliary masks of tissue and epithelium were generated by deep learning classifiers as heatmaps. (B) The masked heatmaps were then grided into different sub-region scales (spatial pyramid pooling levels) and different statistic values were adopted as EPFs.
Results
Four EPFs of G4_min, S4_median, L6_min and N4_min were selected. The Spearman test showed no correlationship between each 2 selected EPFs. The Harrell concordance index of the Cox model were 0.61 and 0.65 in discovery dataset, where the model was developed, and external validation dataset, respectively. All selected EPFs performed as risk factors in Cox model. The forest plot of the Cox model and projected EPFs can be found in Fig.2, where these EPFs can be easily recognized visually.
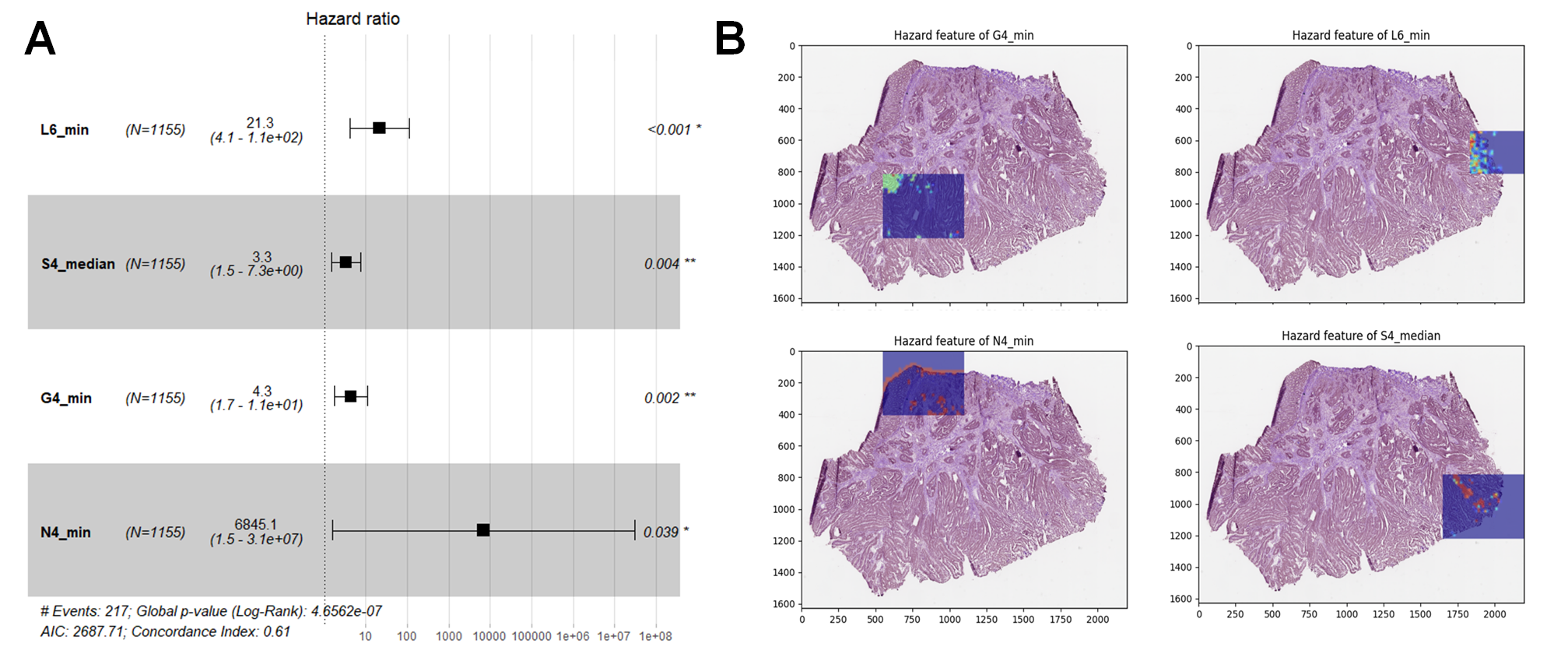
Fig.2 (A) The forest plot of the Cox model on selected EPFs. (B) The projection of G4_min, L6_min, N4_min and S4_median EPFs back to the original WSI. For G4_min, the color in the heatmap was closer to red referred to lower gland formation. For the other 3 EPFs, the color was closer to red referred to higher probability of related visual characteristics.
Conclusion
Our approach not only built a robust model to predict survival outcomes but also help to reveal valuable clues of pathology through EPFs’ interpretability. For example, the G4_min indicated that the sub-region with highest tumor gland formation, which can be considered as the shortest stave of Liebig’s barrel, decided the risk of the WSI.