Is one contour all we need? Rethinking the output of DL tumour auto-segmentation models for OPC
Alessia De Biase,
The Netherlands
MO-0800
Abstract
Is one contour all we need? Rethinking the output of DL tumour auto-segmentation models for OPC
Authors: Alessia De Biase1, Nanna Maria Sijtsema1, Lisanne van Dijk1, Roel Steenbakkers1, Johannes Langendijk1, Peter van Ooijen1,2
1UMCG, Radiation Oncology, Groningen, The Netherlands; 2UMCG, Data Science Centre in Health (DASH), Groningen, The Netherlands
Show Affiliations
Hide Affiliations
Purpose or Objective
Currently, the quality of Deep Learning (DL) generated organ at risk (OAR) contours is acceptable for clinical use in most cases. However, auto-segmentation of tumours using DL is still a challenge. One potential explanation is the inter-patient variability in tumour locations and imaging characteristics. We estimated the uncertainty related to this variability by training models on different patient subsets by cross-validation (CV) and then averaging the multiple models output in a final prediction. As a result, the range of the predicted pixel values is widened and the output looks like a probability map where high probability areas correspond to higher (and low probability areas to lower) agreement among trained models. It is this information that we would like to present to radiation oncologists as a starting point in the tumour contouring process. In this study, we aim to demonstrate that in order to obtain optimal generated GTVp (Gross Tumour Volume of the primary tumour) contours it is necessary to rethink the output of DL tumour auto-segmentation models taking into account model uncertainty.
Material and Methods
Planning PET-CT and GTVp contours of 301 oropharyngeal cancer (OPC) patients treated with (chemo)radiation from 2014 to 2022 in our institute were collected. We used 241 patients to perform 3-fold CV and 60 patients to test the DL network for tumour segmentation. Each voxel value of the model output represents a tumour probability (Figure 1-right column). To assess the model performance, surface dice similarity coefficients (surface-DSC) with the GTVp contours were calculated for different probability thresholds. For each patient, the optimal threshold was assessed with the highest value of surface-DSC. Finally, patients were grouped according to their optimal probability thresholds and the groups’ s were determined.
Results
The average surface-DSC in the test set ranged between 0.34 and 0.77, showing an increasing pattern across thresholds. Figure 1 shows that using the probability map for three different patients, the optimal tumour contour is based on different probability thresholds. In Figure 2, a barplot is used to quantify this variability. Selection of the most frequent threshold would only be optimal for 40% of the patients of the test set. Thus, there is not one optimal probability threshold for all cases. It would therefore be better not to select a single threshold but to offer the radiation oncologist contours for different threshold values so that the most suitable one for individual patients could be used as a starting point for tumour contouring. Furthermore, each voxel value could give additional information about the spatial uncertainty in predicted tumour contours.
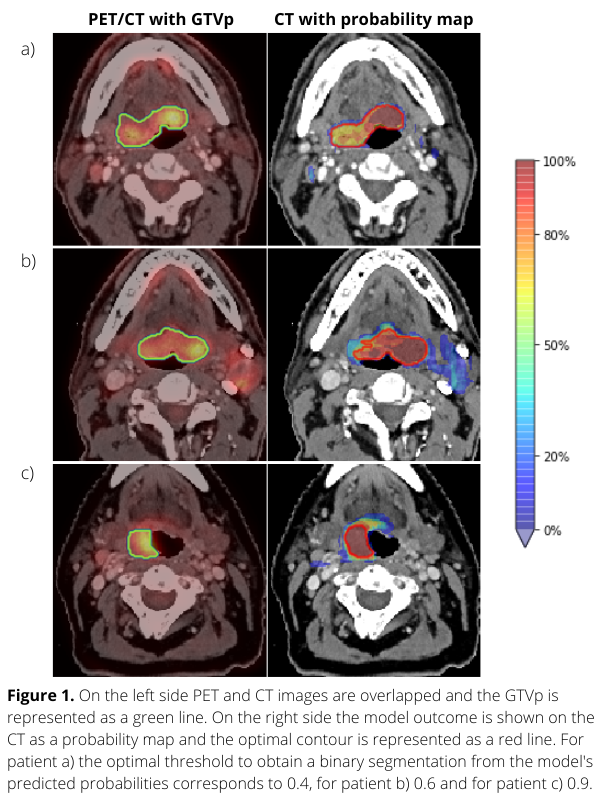
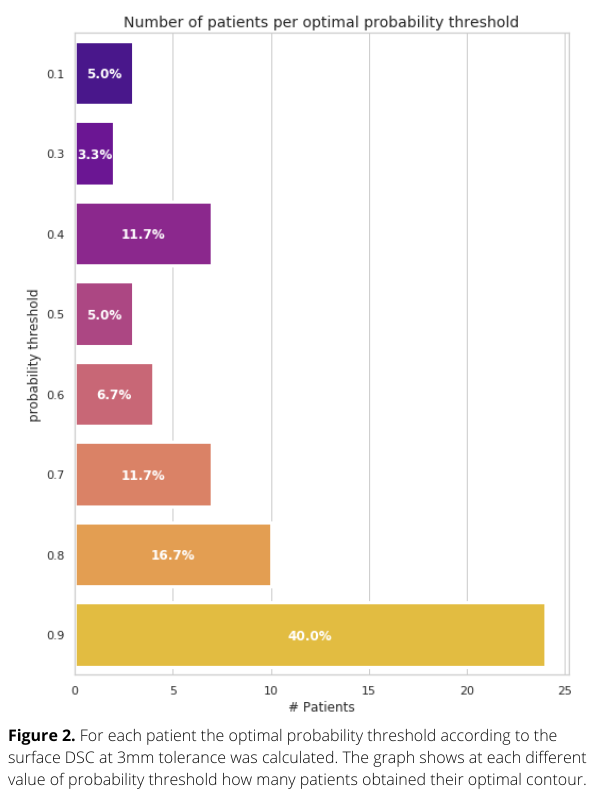
Conclusion
Presenting the tumour probability map with adjustable probability thresholds as output from the DL tumour contouring model gives clinically useful additional information for the radiation oncologist that can be used to optimize the tumour contouring process.