Evaluation of target autocontouring for MRI-guided online adaptive treatment of rectal cancer
Nicole Ferreira Silverio,
The Netherlands
MO-0798
Abstract
Evaluation of target autocontouring for MRI-guided online adaptive treatment of rectal cancer
Authors: Nicole Ferreira Silverio1, Wouter van den Wollenberg1, Anja Betgen1, Lisa Wiersema1, Corrie Marijnen1, Femke Peters1, Uulke A. van der Heide1, Rita Simoes1, Tomas Janssen1
1The Netherlands Cancer Institute, Radiation Oncology, Amsterdam, The Netherlands
Show Affiliations
Hide Affiliations
Purpose or Objective
Online re-delineation of the clinical target volume (CTV) for MRI-guided online adaptive radiotherapy of rectal cancer allows the reduction of treatment margins. However, re-delineation is time consuming and logistically challenging. Deep Learning (DL) based auto-contouring could improve the process, but validation of autocontouring of the CTV requires careful consideration before clinical introduction. The aim of this work is to present and apply a comprehensive commissioning framework for DL autocontouring of CTVs.
Material and Methods
We included rectal cancer patients treated on the Unity MR-Linac (Elekta AB, Stockholm) with 5x5 Gy. An in-house trained DL auto-contouring model for the mesorectum CTV was available. During development the model was evaluated on geometrical metrics and results were discussed with experts. Evaluation of the final model prior to clinical introductio n was done according to the following steps
- Inter-observer variation (IOV) was determined by having 5 clinicians delineate the CTV on 10 scans (dataset 1). IOV was quantified using the Dice, surface Dice en 95% Hausdorff distance.
- The predicted CTV (CTVpred) was compared with the manually delineated CTV (CTVman) using the same geometric metrics (dataset 2: 77 scans).
- One clinician corrected CTVpred to a clinically acceptable delineation CTVcor (dataset 3: 49 scans). CTVpred was scored on a scale of 0-4 (0 = no corrections, 4 = unusable). Time spent correcting was measured. CTVpred and CTVcor were compared on geometric metrics and benchmarked against the results from steps (1) and (2).
- We determined the overlap between PTVpred and PTVcor (pre-treatment) with independent CTVman (post-treatment) (using dataset 3). Overlap was averaged over all available treatment fractions (2-5 fractions) for different CTV-PTV margins. Per margin, we report the coverage reached by 90% of the population.
Results
Table 1 shows the result of the geometric metrics (step 1-3) of the evaluation. We found that while there is a difference between CTVpred and CTVman (column 2), this difference is comparable to the IOV (column 1) and only minor changes are required to CTVpred (column 3). Corrections were mainly required caudally, corresponding to the region with the largest IOV.
Figure 1 shows the achieved post-treatment target coverage, showing that after correction target coverage is higher, indicating the potential clinical relevance of the corrections.
Average correction time was 2.4 minutes (compared to current clinical practice of 7 minutes). Two CTVpred were discarded as unusable and nine predictions needed no correction.
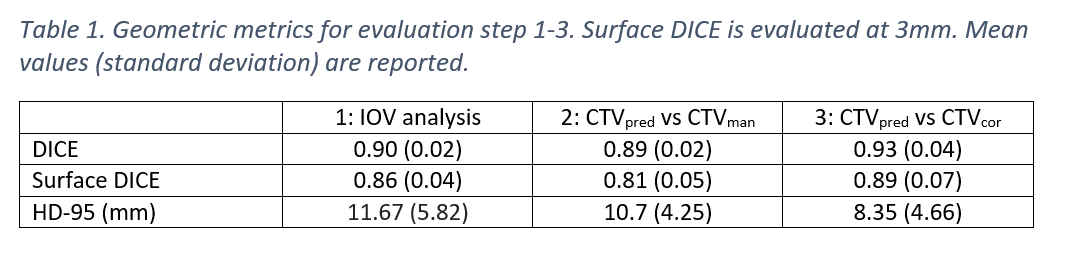
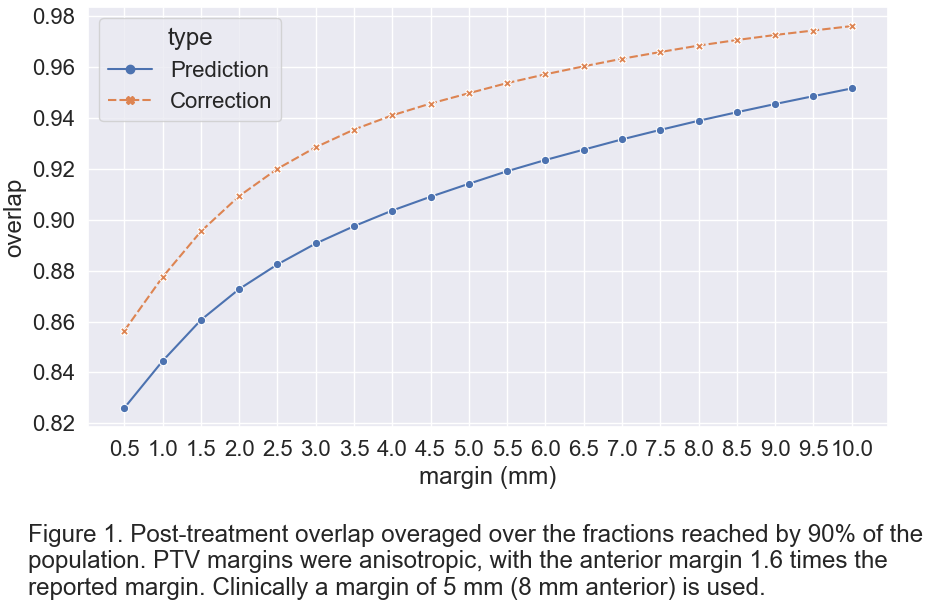
Conclusion
We present and apply a framework that allows the evaluation of DL autocontouring of the CTV. Model performance was comparable to the IOV and minor changes were required in regions of significant IOV. We showed the potential clinical relevance of the corrections, since target coverage increased after correcting. These findings combined allow the safe clinical commissioning of a DL model for CTV autocontouring.