Implementation of direct quality of life guided treatment plan optimisation
Arjen van der Schaaf,
The Netherlands
OC-0181
Abstract
Implementation of direct quality of life guided treatment plan optimisation
Authors: Arjen van der Schaaf1, Hans Paul van der Laan1, Lisa Van den Bosch1, Erik Korevaar2, Stefan Both1, Johannes A. Langendijk1
1University of Groningen, University Medical Center Groningen, Radiation Oncology, Groningen, The Netherlands; 2University of Groningen, University Medical Center Groningen, Radiation Oncology , Groningen, The Netherlands
Show Affiliations
Hide Affiliations
Purpose or Objective
Conventional radiation treatment planning strategies intend to maximise survival and quality of life (QoL) of cancer patients but are based on subjective interpretation of how QoL is related to the dose distribution. We aim to implement a closed loop between observed QoL and treatment plan optimisation and reduce the need of human interaction.
Material and Methods
Prospective data of 750 patients treated for head and neck cancer were analysed to find NTCP models for 20 common toxicities at 6 to 24 months after treatment. Subsequently, a linear relation between those toxicities and QoL, defined as an average of six EORTC QLQ-C30 items, was fitted with use of dimensionality reduction (PCA) and resulted in a weight factor of each toxicity with respect to QoL. The NTCP models and their weights were then used in treatment plan optimisation. Models were programmed into the optimiser of RayStation using C++ and the Raysearch Research functions API with adaptations to make the functions convex and smooth to ensure convergence. Case specific settings of the objective functions were prepared in a spread sheet and imported in RayStation with a python script. Objectives for target coverage, hot spots, and conformity were manually adjusted iteratively until the plan was clinically acceptable (see figure). The planning study was conducted with 31 patients.
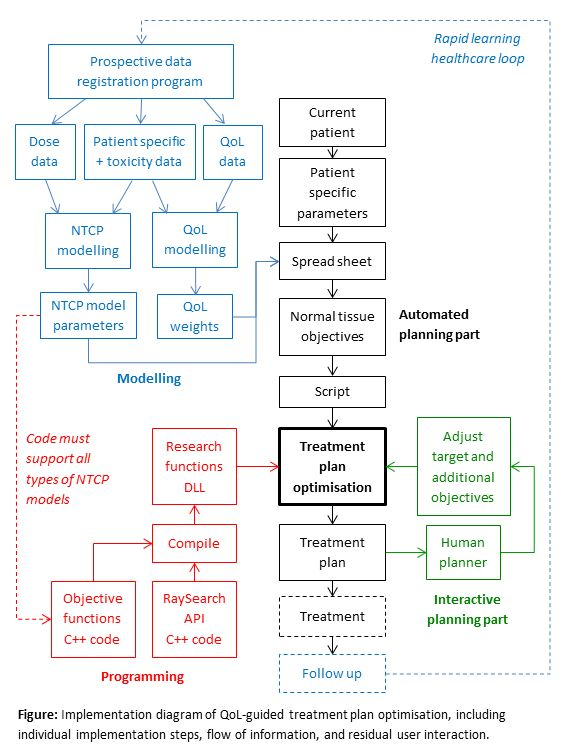
Results
QoL-guided optimisation converged properly and planning required less human interaction than with conventional planning. QoL-guided plans showed improved predicted QoL with equal target coverage. The improvement was achieved largely by decreased sparing of the parotid glands and increased sparing of other normal tissues (see table). This can be explained because the parotid glands were historically the first organs to be actively spared and have retained a very high priority in conventional planning, while this priority seems unjustified in QoL-guided optimisation.
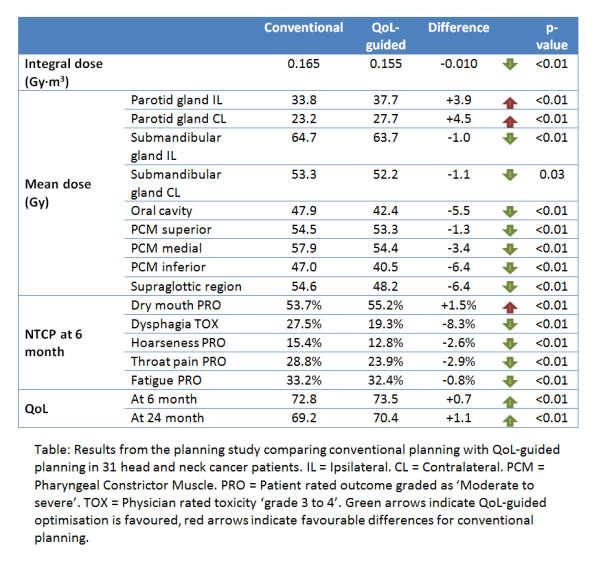
Conclusion
We have shown that direct QoL-guided plan optimisation is feasible, improves expected QoL, and facilitates planning process automation. In the current implementation human interaction is still needed to tune target coverage, hot spots, and dose conformity, but adding models for these clinical goals in relation to QoL would render the process fully automated. Moreover, the automation is not based on mimicking human planning (such as with AI solutions) but on modelling of historic patient data, which can be continuously updated in a closed rapid learning healthcare loop. This process translates observed outcomes directly and objectively into optimal planning strategies whereas conventionally this process involves subjective human interpretation or historic habits. Future developments are needed to support more generic models with less scripting and C++ programming and to allow non-convex objective functions.