Lambri N, Dei D, Goretti G, Crespi L, Coimbra Brioso R, Pelizzoli M, et al.
Physics and Imaging in Radiation Oncology. 2024;31:100617. doi: 10.1016/j.phro.2024.100617.
Interview with Pietro Mancosu, last and correspondence author
What was your motivation for initiating this study?
Currently, most radiotherapy plans are delivered through the use of intensity-modulated techniques. This study was initiated to address the challenges posed by complex radiotherapy plans, which are associated with greater uncertainties and potential failures in patient-specific quality assurance (PSQA). Although technological advances mean that only a small fraction of these plans fail PSQA, such failures still pose risks to patient safety. Therefore, it is crucial to explore new approaches that minimise these risks. This work is part of Nicola Lambri’s PhD dissertation, first author of the paper.
Our idea was to leverage the extensive data and experience that had been acquired over many years and to integrate them with Lean Six Sigma methodology. Lean is focused on the elimination of inefficiencies, while Six Sigma is designed to reduce process variability and defects. Used together, these methodologies streamline processes and improve quality. Additionally, the traditional measurement-based PSQA process is resource-intensive, which can slow clinical workflows. The integration of machine learning (ML) and Lean Six Sigma was intended to show which plans could be at risk of failure before they were used. This would enable us to intervene before PSQA measurements were taken, and thus to speed up the process.
Our objectives were twofold: first, to monitor outlier complexity and enhance the overall quality of radiotherapy plans while ensuring that they still met clinical goals; and second, to reduce the risk of PSQA failure and improve the efficient allocation of resources, which would enable less complex plans to undergo a streamlined QA process that required fewer time-consuming measurements.
• What were the main challenges during the work?
One of the main challenges was to develop an ML model that could accurately predict the gamma passing rate (GPR) for radiotherapy plans, particularly in datasets in which only a small number of plans exhibited low accuracy. Ensuring high prediction accuracy while minimising the number of false positives required a careful balance between the capabilities of artificial intelligence (AI) and human oversight.
Another challenge was the bringing together of expertise from various fields, such as medical physics, AI and clinical practice, to develop a robust model. The use of the define, measure, analyse, improve, control approach, which is taught by the Lean Six Sigma methodology, was instrumental in providing a structured framework for the optimisation and control of the complex radiotherapy planning and PSQA processes. Interaction with the Lean group and in particular with Dr Giulia Goretti was fundamental to the success of this study.
• What is the most important finding of your study?
The most significant finding is that the use of ML, when combined with Lean Six Sigma, can create a highly effective, targeted PSQA approach that improves both the quality and safety of radiotherapy plans. Through our use of AI guided by human intelligence, we were able to identify plans that were at risk of failure (i.e., outliers) and reduce their number. Additionally, the median GPR improved from 97.4% to 98.2%.
This study underscored the importance of multidisciplinary collaboration to achieve successful outcomes. The combined expertise of medical physicists, radiation oncologists, AI specialists and quality experts was crucial to ensure the robustness of the entire process.
What are the implications of this research?
This research highlights the pivotal role of multidisciplinary collaboration in modern healthcare; it shows how medical physicists, engineers, clinicians, and quality managers can work together to enhance the efficiency and safety of radiotherapy. Through the incorporation of Lean methodology for continuous quality improvement, the project demonstrates how streamlined workflows can improve patient safety.
Furthermore, the findings underscore that the use of AI, when combined with human expertise, can produce better-targeted QA processes, reduce clinical workload, and improve the accuracy of radiotherapy plans.
This work is part of a larger project that is intended to support virtual PSQA with a focus on uncertainty estimation and enhanced quality management. It involves experts from Humanitas University, the University of Milan (Professor Cristina Lenardi), Politecnico di Milano (Prof Daniele Loiacono), and University College London (Prof Andy Nisbet). The involvement of enthusiastic students from these institutions has been crucial to the success of the initiative.
Pietro Mancosu, PhD
Humanitas University and IRCCS Humanitas Research Hospital, Rozzano (Milan), Italy
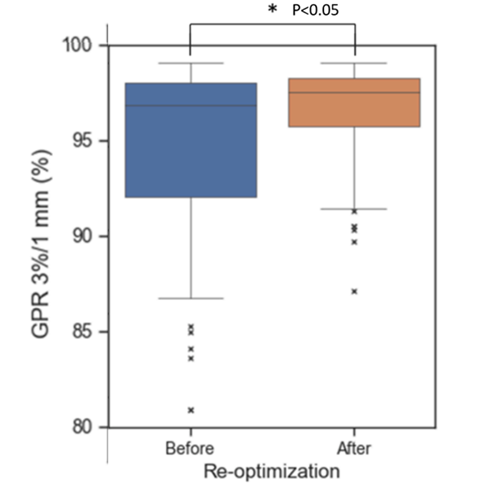