Statistical resources for medical physics and beyond - PDF Version
As two PhD researchers in medical physics who have an active interest in statistical modelling, most weeks at our workplace involved a tea-room chat about statistics, or a seminar from experts outside the radiotherapy field. With last year's switch to working from home, we started a search for content that was freely available online to get this same experience. The resources we found should serve as a useful guide to researchers, at any stage of their careers, who are looking to brush up on a few skills or adopt a whole new approach to thinking about their research problem.
The best way to start with finding expert help at the touch of a button is with Professor Frank E. Harrell Jr. of Vanderbilt University. With his course in biostatistics for biomedical research, Prof Harrell becomes the statistics professor you always wanted in the comfort of your own home.1 The course notes take you on a journey from a basic algebra review to complex statistical problems that we typically experience in the medical physics world, such as high-dimensional data or observational treatment comparisons. Alongside the course notes, there are opportunities to engage in the lectures in real time, and the first 15 one-hour-long lectures are available on the course YouTube channel. This course is accessible to a beginner, and has an additional recommended reading list for those with no statistics training. However, even more experienced modellers can gain insight from Prof Harrell’s course, as he is not afraid to call out common misunderstandings in medical literature. With almost 600 pages of notes and plenty of options for additional reading, this course can require a big investment of time from busy researchers - but it’s worth it for improved confidence in future analysis.
Prof Harrell also finds the time to be actively engaged on Twitter (@f2harrell) and to update regularly his blog, Statistical Thinking. This is ideal for anyone who wants quick access to new techniques.2 Some of our favourite blog posts include: ‘Statistically efficient ways to quantify added predictive value of new measurements’, which should be read by everyone who is involved in biomarker research; and ‘Improving research through safer learning from data’, which offers important tips for both descriptive and inferential analyses. If the articles leave you with more questions than answers about how to adopt these approaches, there is a dedicated ‘Datamethods’ discussion forum where you can ask your questions and receive friendly advice. No question that is posed there seems to be missed by Prof Harrell himself.3
For those interested in building and validating prognostic models, Professor Richard Riley and Dr Kym Snell at Keele University have set up a website on prognosis research to collate useful resources with an “aim to improve prognosis research in healthcare”.4 When we attended the incredibly useful course entitled ‘Statistical methods for risk prediction and prognostic models’ at Keele University in 2019, our eyes were opened to the common pitfalls that occur in prognosis research, whilst we were given the knowledge and tools to overcome them. The website is a free resource that gives you a taste of the course itself. Recorded talks are available to help answer questions such as ‘what sample size do I need?’. As this is a new resource, we look forward to finding out what content will be added next.
If you are missing the feelings associated with attendance at seminars, we advise that you closely follow the virtual events posted by the Royal Statistical Society (RSS), although croissants and bad coffee are unfortunately not included. As students, we have the opportunity to attend many workshops and seminars for free, and there is a series of public lectures available on the RSS YouTube channel.5 Highlights of this include the ‘Interpretable machine learning and causal inference workshop’, where we have enthusiastically heard Dr Peter Tennant from Leeds University provide us all with a warning regarding inappropriate interpretation of model coefficients. He advises viewers that a causal understanding should be obtained for true interpretability.
Causal inference is another topic in itself - I’m sure many of us have heard the mantra ‘correlation does not imply causation’. A fantastic introduction to this topic is given by Professor Miguel HernĂ¡n of Harvard University via HarvardX.6 Through this route, you can learn via real-life examples how causal diagrams can help to identify confounders and casual effects. This course requires only two to three hours a week of effort over nine weeks, and is perfect for beginners.
This year might also be the one in which you decide to transition from a frequentist to a Bayesian approach, an alternative statistical approach to traditional null-hypothesis testing in which p-values do not exist. The course entitled ‘Statistical rethinking’ by Professor Richard McElreath of Leipzig University is an excellent resource to introduce the world of Bayesian statistics. Prof McElreath’s unique story-telling style is engaging and enables readers to grasp the basic concepts before learning how to develop more complex models. Helpfully, coding examples are given in both R and Stan, so you can practice the concepts as you learn them. His course lectures are available via YouTube and include 20 one-hour-long lectures that follow his book.7 A basic knowledge of regression modelling is all that’s required to understand the course, and experience in R if you want to follow the coding examples. Advantages of the Bayesian approach include the lack of a requirement to correct for multiple hypothesis testing and models that allow incorporation of past data. Prof Harrell has written many interesting blog articles on Bayesian statistics, including ‘My journey from frequentist to Bayesian statistics” and ‘Continuous learning from data: no multiplicities from computing and using Bayesian posterior probabilities as often as desired’.
We hope these suggested statistical resources are helpful and look forward to seeing your faces virtually at some of the upcoming statistical workshops soon.
Isabella Fornacon-Wood and Angela Davey
PhD researchers, The University of Manchester, UK
References:
[1] https://hbiostat.org/bbr/
[2] https://www.fharrell.com/
[3] https://discourse.datamethods.org/
[4] https://www.prognosisresearch.com/
[5] https://www.youtube.com/user/RoyalStatSoc
[6] https://www.edx.org/course/causal-diagrams-draw-your-assumptions-before-your
[7] https://xcelab.net/rm/statistical-rethinking/
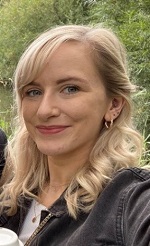
Angela Davey
angela.davey@manchester.ac.uk
@AngieDavey
Angela Davey is a final-year PhD researcher in the adaptive radiotherapy group at The University of Manchester (@RTPhysics). She is working on the identification of patient characteristics for personalisation of radiotherapy in lung cancer treatment with Dr Alan McWilliam, Professor Marcel van Herk, and Professor Corinne Faivre-Finn. As part of her PhD, she uses radiomics and image-based data-mining to explore the interactions between peritumoural density and delivered dose to predict recurrence in mobile lung tumours that are treated with stereotactic body radiation therapy (SBRT). She obtained her master of physics degree at The University of Manchester.
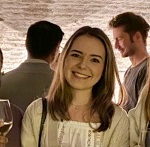
Isabella Fornacon-Wood
isabella.fornacon-wood@postgrad.manchester.ac.uk
@Isabellamfw
Isabella Fornacon-Wood is a third year PhD researcher in the adaptive radiotherapy group at The University of Manchester (@RTPhysics). She is currently investigating methods that can be used for rapid analysis of healthcare data to evaluate changes in radiotherapy protocols with Dr Gareth Price, Professor Corinne Faivre-Finn and Professor James O’Connor. She obtained a bachelor of physics degree at Leipzig University and a masters of research in translational medicine at The University of Manchester.