ESTRO 2024 Congress report
The accurate delineation of target volumes in radiotherapy has long been a critical challenge, especially in the complex anatomy of head-and-neck cancer. Traditional methods of manual delineation of gross tumour volume (GTV) suffer from significant variability and inaccuracies across different experts and healthcare facilities, and these issues often lead to over-irradiation of healthy tissues, insufficient tumour control and disease recurrence. Our recent study offers a groundbreaking solution to this issue by harnessing the power of histopathology and advanced deep-learning models.
Our approach leverages digitised histopathology slides, which provide a gold standard for tumour characterisation, to inform and enhance the accuracy of CT-based target volume delineation. Through the development of a sophisticated multimodal registration method that is driven by artificial intelligence, we were able to transfer precise tumour contours from histology to CT images. This novel method addresses the deformations and tissue changes that occur during the histological process and ensures accurate mapping between the two modalities.
Next, we used these histological contours of tumours mapped onto CT images and developed a state-of-the-art segmentation model. These high-quality annotations can be used to unlock the potential of deep learning for automatic target volume delineation, something that was previously impossible due to the variability and inaccuracy of manual GTV.
More precisely, the culmination of our segmentation pipeline was achieved through the use of an advanced technique known as diffusion modelling. Instead of just providing a simple outline of the tumour, it generates a map that shows areas with varying levels of uncertainty about the presence of the tumour. This provides a much clearer and more detailed picture of the tumour's extent and its variations. This approach is a significant improvement over traditional methods, allowing for more accurate and personalised radiotherapy planning that is tailored to the unique characteristics of each patient's tumour.
The implications for clinical practice are substantial. Our histology-enhanced GTV method reduces the reliance on manual delineation, thus minimising the risk of human error and variability. This leads to more consistent and accurate treatment plans than were possible before, potentially improving patient outcomes. Additionally, the ability to quantify uncertainties in the segmentation process provides clinicians with valuable insights into the reliability of the delineations, which facilitates more informed decision-making in treatment planning.
Looking ahead, the potential for end-to-end clinical translation of our method is exciting. We are exploring avenues for future development. These include dose prediction models, which are possible through the extension of our probabilistic GTVs into probabilistic clinical target volumes and planning target volumes. This change would enable more precise dose delivery and pave the way for dose painting. Furthermore, we are investigating the use of immunohistochemistry on digitised slides to highlight specific tumour biomarkers, which could add another layer of detail to our segmentation models and enhance the characterisation of tumour heterogeneity.
In summary, the integration of histological data into radiotherapy planning through the use of advanced deep-learning models marks a significant advancement in the field. Our study offers a fresh perspective on target volume delineation, emphasising its potential to improve clinical outcomes and foster the development of personalised treatment strategies. The journey from this research to clinical application promises to transform the landscape of radiotherapy and to benefit patients with more accurate and individualised treatment options.
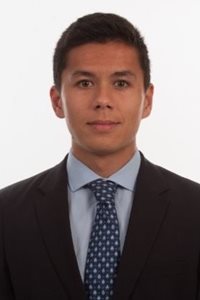
Amaury Leroy
Therapanacea, Paris, France
Institut Gustave Roussy, Villejuif, France
Centre Léon Bérard, Lyon, France
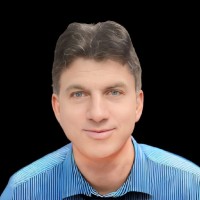
Nikos Paragios
Therapanacea, Paris, France