Generating synthetic computed tomography for radiotherapy: a look at the SynthRAD2023 challenge
ESTRO 2024 Congress report
Introduction
Radiotherapy plays a critical role in cancer treatment Radiotherapists aim to deliver a precise dose of radiation to tumours while minimising damage to healthy tissues. However, accurate determination of patient anatomy for radiotherapy planning can be challenging, especially considering that daily variations are captured on cone beam CT (CBCT) that require treatment adaptation. Additionally, the replacement of CT with MRI-only approaches during patient simulation would be desirable to avoid exposure of patients to imaging radiation. However, MRI lacks the electron density maps that are crucial for treatment planning.
These challenges have fuelled the development of ways to generate synthetic CT (sCT) scans from MRI or CBCT (Spadea et al., 2020).
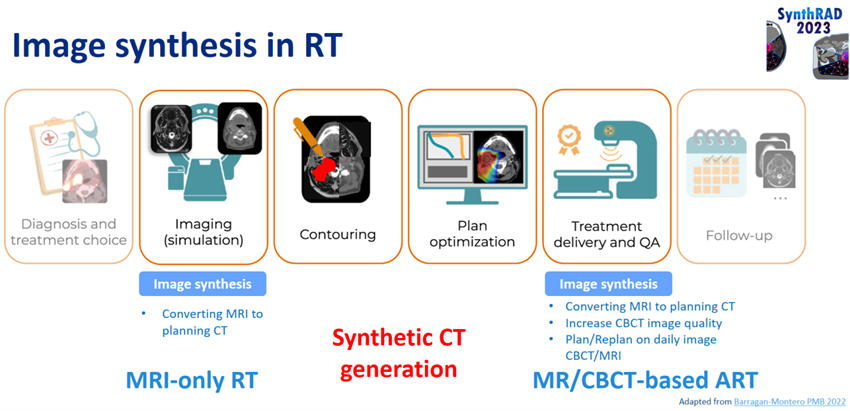
SynthRAD2023 Grand Challenge: Fostering Innovation in sCT
The SynthRAD2023 Grand Challenge (https://synthrad2023.grand-challenge.org/) was designed to accelerate advancements in sCT generation. Participants focused on developing fully automated sCT algorithms. The challenge involved the performance of two tasks:
- Task 1: MRI-to-CT generation for MRI-only radiotherapy.
- Task 2: CBCT-to-CT generation for adaptive radiotherapy.
The publicly available challenge dataset comprised paired MRI/CT or CBCT/CT scans from over 1080 pelvis and brain cancer patients who had been treated at three Dutch university medical centres (Thummerer et al., 2023). The dataset was split into training, validation, and test sets for each task, in order to ensure balanced representation from all three centres.
Participants received training data and validation input to refine their algorithms and to submit generated sCTs. These submissions were evaluated for image similarity to CT.
Participant algorithms were run on the hosting platform during the test phase to generate sCTs. Using open-source software, these sCTs were then used to recalculate previously optimised photon and proton treatment plans. The accuracy of sCT-based dose calculations was assessed using dose differences, dose-volume histogram-based metrics, and gamma pass rate analysis against the CT-derived dose distributions.
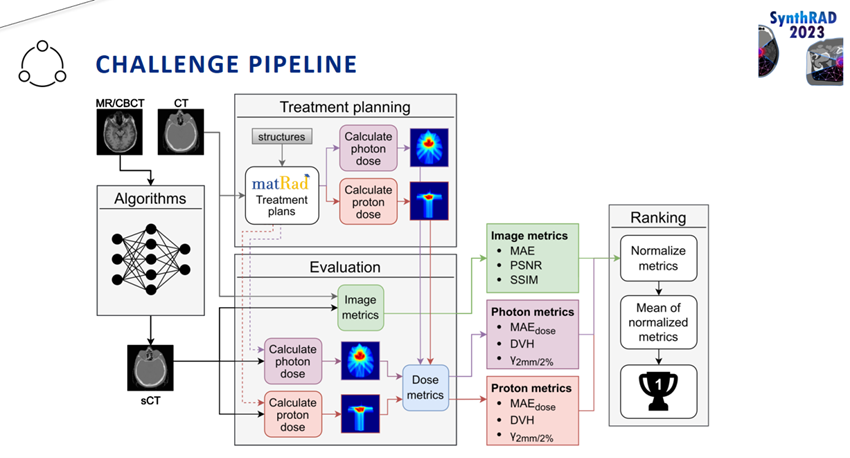
Key Findings and Future Directions
The challenge attracted significant interest, with over 600 registrations and 40 final valid submissions (Huijben et al., 2024). One team achieved the highest scores in both tasks, and the sCT of the top-performing teams resulted in high image similarity and minimal dose deviations compared with the original CT-based plans. Interestingly, no clear correlation was found between specific algorithm architecture and performance.
The post-challenge phase of SynthRAD2023 is still open, to enable continuous evaluation of new algorithms.
The challenge highlighted that image similarity alone cannot guarantee accurate dose calculations. While some submissions achieved strong similarities in the scores for images, their corresponding dose calculations deviated from the original CT-based plans. This finding emphasises the importance of integrating clinical dose evaluation alongside image similarity metrics for a comprehensive assessment of sCT solutions.
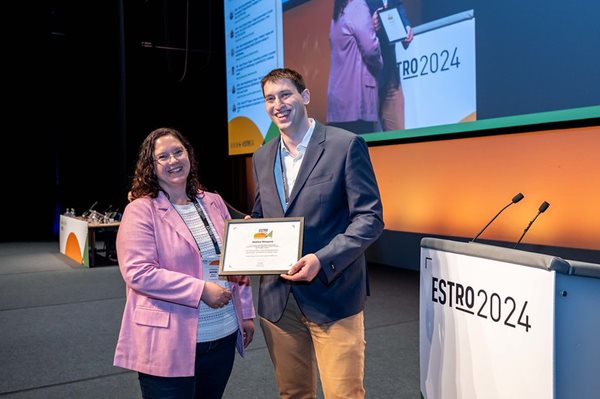
Matteo Maspero receiving the Best Physics Paper award at ESTRO 2024, with Monica Buijs, Co-chair of the session
Conclusion
The SynthRAD2023 Grand Challenge successfully provided a platform for researchers to develop and benchmark sCT generation techniques. The competition offered valuable insights into the development of MRI-only and CBCT-based adaptive radiotherapy algorithms.
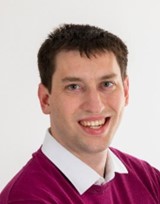
Matteo Maspero, on behalf of the SynthRAD2023 organisers
Department of Radiotherapy,
University Medical Centre (UMC) Utrecht,
Utrecht, The Netherlands
Matteo Maspero
Corresponding author
Authors
Evi Huijben1, Maarten Terpstra2,3 , Arthur Jr. Galapon4, Suraj Pai5, Adrian Thummerer4,11, Peter Koopmans6, Manya Afonso7, Maureen van Eijnatten1, Oliver Gurney-Champion8,9, Zoltan Perko10, Matteo Maspero2,3 (corresponding)
1 Department of Biomedical Engineering, Eindhoven University of Technology, Eindhoven, The Netherlands
2 Department of Radiotherapy, UMC Utrecht, Utrecht, The Netherlands
3 Computational Imaging Group for MR Diagnostics & Therapy, UMC Utrecht, Utrecht, The Netherlands
4Department of Radiation Oncology, UMC Groningen, Groningen, The Netherlands
5Department of Radiation Oncology, GROW School for Oncology, Maastricht University Medical Centre, Maastricht, The Netherlands
6Department of Radiation Oncology, Radboud UMC, Nijmegen, The Netherlands
7Wageningen University & Research, Wageningen Plant Research, Wageningen, The Netherlands;
8Department of Radiology and Nuclear Medicine, Amsterdam UMC, University of Amsterdam, Amsterdam, The Netherlands
9Cancer Center Amsterdam, Imaging and Biomarkers, Amsterdam, The Netherlands
10Delft University of Technology, Faculty of Applied Sciences, Department of Radiation Science and Technology, Delft, The Netherlands
11Department of Radiation Oncology, LMU University Hospital, LMU Munich, Munich, Germany
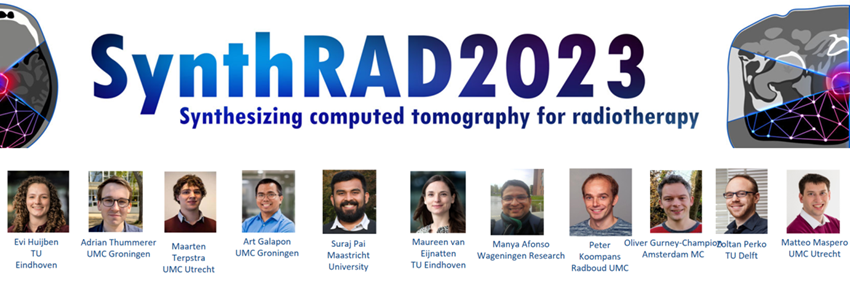
References
1 Spadea MF, Maspero M, Zaffino P, Seco J. (2021). Deep learning-based synthetic-CT generation in radiotherapy and PET: A review. Medical Physics, 48(11), 6537–6566. https://doi.org/10.1002/mp.15150
2 Thummerer A, van der Bijl E, Galapon Jr A, Verhoeff JJ, Langendijk JA, Both S, van den Berg CAT, Maspero M. (2023). SynthRAD2023 Grand Challenge dataset: Generating synthetic CT for radiotherapy. Med Phys 2023;1-11. https://doi.org/10.1002/mp.16529
Huijben E, Terpstra LM, et al. Generating Synthetic Computed Tomography for Radiotherapy: SynthRAD2023 Challenge Report. Mar 2024, https://doi.org/10.48550/arXiv.2403.08447.